How AI/ML Ensures Data Quality to Drive Enterprise Excellence
An opinion piece by the head of enterprise data science at Capital One
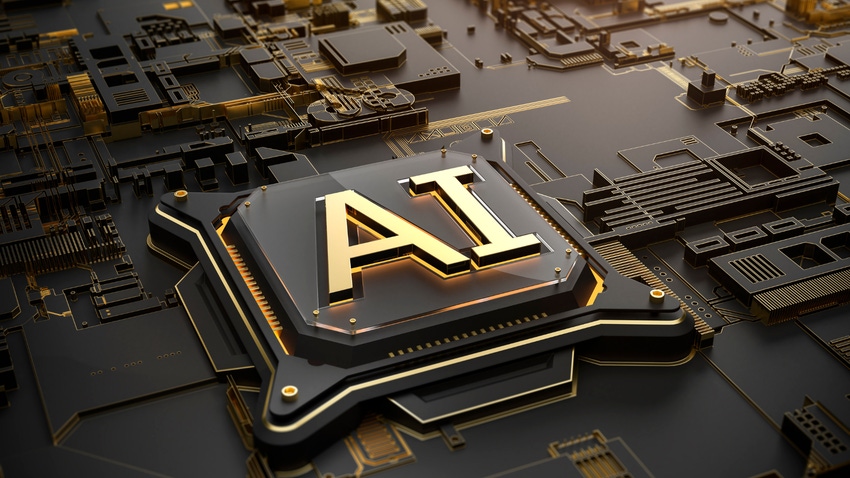
The magic behind many of today’s most lauded digital customer experiences − like the streaming music service that can match your exact mood or the powerful chatbot that can make you an organized grocery list for the week — is often broadly attributed to AI.
But AI would not be able to do any of that without the real workhorse behind the magic: data. Delivering great digital products and experiences today requires high-volume, high-quality input data. For example, all of the recent progress in large language models is due to using more − and better − data on a large scale.
But ensuring the safe and secure use of high-quality input data is deeply complex. The amount of data generated globally has exploded by an estimated 60x − from 2 zettabytes since 2010. (A zettabyte is equal to 1 billion terabytes).
Analysts, data scientists, and ML engineers are increasingly overwhelmed by a constant flood of inaccurate, incomplete, outdated, inconsistent, and redundant data. Cutting across an enterprise’s silos to collect, clean, protect, and access all of its data is also incredibly difficult. And data quality challenges will continue to grow as new sources and formats emerge.
A recent Great Expectations survey found that nearly eight out of 10 data professionals experience data quality issues, and 91% of those believe that these problems are hurting company performance. Gartner says that poor data quality costs organizations $12.9 million annually, on average.
Ensuring data stays high quality while being well-managed throughout its lifecycle requires the ability to spot and resolve issues quickly. Identifying and correcting data quality issues is time-consuming, costly, and takes very specific subject matter expertise. According to a Monte Carlo and Wakefield Research survey, 75% of data professionals say it takes four or more hours to detect data quality issues and about nine hours to resolve them.
Using AI/ML
Fortunately, organizations have opportunities at every touchpoint of the data lifecycle to focus on improving their data quality to drive better user experiences. One best practice is to apply automation in the form of machine learning tools and applications to the problem. According to a recent Forrester Consulting study commissioned by Capital One, 53% of data professionals plan to improve business efficiency with ML applications.
Automation and standardization through ML allows tech teams to tackle the sheer size and complexity of data quality and governance tasks in ways that manual, rote processes could not handle nearly as efficiently or effectively.
A good example of applying ML to data quality control is the automatic detection of sensitive data within large datasets, monitoring how the data is being used, and prompting data producers to take action immediately when issues are detected. This can help keep sensitive data protected and secure.
By automating the detection of data-related issues, organizations can more precisely, actively, and even proactively address data quality issues before or as they arise.
We built and open-sourced our own tool called Data Profiler at Capital One to detect sensitive data across large batches of data (distribution, pattern, type, and so forth). It helps perform many tasks including staff optimization, pushing out rules at scale, intelligent monitoring and alerts, and root cause analysis.
Combating model drift
Monitoring for drift is another essential practice that can be automated with ML tools. Drift is a common problem with models built on historical data. Data is not static; it constantly changes, like every person and the broader world around us. Relying too heavily on historical data can generate counterintuitive estimates or less accurate predictions over time, broadly known as model drift.
One way to combat model drift is through real-time adaptation. An intelligent assistant interacting with customers on a website can feed those interactions back to its ML models to better predict what customers need or want.
For example, a customer switching over to our credit score platform, CreditWise, for a few minutes after looking at their checking account is a data point that can help fine-tune recommendations about when to suggest solutions for better budgeting and spending.
Real-time adaptability takes time and work, requiring sound model frameworks, tools, data patterns, and governance practices. Data science teams must maintain high standards for user privacy, transparency, security, and control.
ML models will only be effective in production if they are running on the correct data, in the right environment, and being applied to the right use case. And that requires a constant focus on serving human needs, expectations, and goals of the user on the other end of the model.
As technological capabilities with AI and ML become more advanced and the world grows more complex, we need to remain focused on the fundamentals – which means putting data quality first.
About the Author(s)
You May Also Like