Databricks adds model registry to MLflow, its all-in-one machine learning toolkit
Databricks adds model registry to MLflow, its all-in-one machine learning toolkit
October 21, 2019
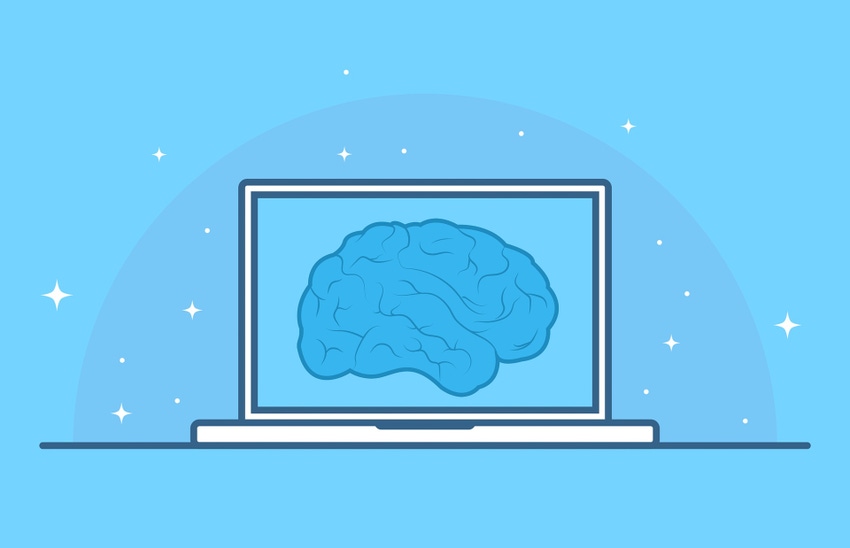
Plus, Delta Lake finds a new home with the Linux Foundation
American startup Databricks, established by the original authors of the Apache Spark framework, continues to bet on AI: this time, it has updated MLflow, the open source machine learning management engine it launched earlier this year.
The latest version of MLflow adds a model registry, so thousands of models can be tracked and shared across the organization simultaneously. The feature also enables closer collaboration between data scientist teams that develop the algorithms, and engineering teams that have to deploy them in production.
Meanwhile, Databricks’ Delta Lake project - designed to take the pain out of storing and processing large datasets - has now been moved under the auspices of the Linux Foundation, world’s largest open source organization.
The announcements were made at the Spark + AI Summit Europe, which took place last week in Amsterdam.
Feeling the flow
MLflow enables data scientists to track and distribute experiments, package and share models across frameworks, and deploy them – no matter if the target environment is a personal laptop or a cloud data center.
The platform supports all popular ML libraries, frameworks and languages, and includes everything required to manage the lifecycle of machine learning projects.
The latest feature to be added is a central machine learning model repository; Databricks says this can help speed up ML deployments and simplify collaboration across development teams.
Among other things, MLflowModel Registry can automatically transition a model into production based on predefined conditions, or manually control and validate lifecycle stage changes from the experimentation phase to testing and production. The platform keeps track of model history and manages who can approve changes.
“Everyone who has tried to do machine learning development knows that it is complex. The ability to manage, version and share models is critical to minimizing confusion as the number of models in experimentation, testing and production phases at any given time can span into the thousands,” said Matei Zaharia, co-founder and CTO at Databricks, and the original author of Apache Spark.
“The new additions in MLflow, developed collaboratively with hundreds of contributors, are enabling organizations worldwide to improve ML development and deployment. With hundreds of thousands of monthly downloads, we are encouraged that the community's contributions are making a positive impact.”
Moving the lake
The Delta Lake project was launched in April to solve the reliability issues plaguing data lakes, those giant repositories of corporate information.
Today, Delta Lakes is being developed by more than 140 contributors and enjoys 800,000 monthly downloads. The core platform is available for free, and Databricks sells a managed version hosted with either AWS or Azure.
Delta Lake is deployed on top of the existing data lake, requiring no change to the underlying architecture. It is compatible with batch and streaming data, can check data quality and schema, and doesn’t allow broken datasets to mess with the algorithms.
From this month, the development of the open source storage layer will be managed by the Linux Foundation. It might have started with Linux, but today LF oversees dozens of software projects, including such staples of modern IT like Kubernetes, Cloud Foundry, Jenkins and Xen.
Databricks hopes that adopting an independent governance model will result in more contributions from the developers, a more active ecosystem, and give Delta Lake more of a chance of becoming a standard for data management at scale.
“Delta Lake has been a proprietary product inside Databricks for over two years; we first open-sourced it in April because we basically felt that if you're going to collect massive amounts of data, you don't want that to be locked into a single-vendor, proprietary black box format,” Michael Armbrust, principal software engineer at Databricks, told AI Business.
“We've always believed that both us and the customers do best when APIs are open, when you can truly move your application from vendor to vendor, and want to stay with Databricks because it's the fastest, cheapest, best place to run - not because the guy who wrote the app left two years ago, and you can't rewrite it.
“I think it's one thing to just slap an open source license on something and say this is a community project. And it's a whole another thing to actually have a vendor-neutral, permanent home for the project, and a governing committee that has written rules about how the project will be run. And the Linux Foundation was just a great home for the project.”
Earlier this month, Databricks announced it would spend €100m over three years to expand its AI lab in Amsterdam.
About the Author(s)
You May Also Like