AI-powered Brain Implants Break Speech Barriers for Paralyzed Patients
The AI technology was found to aid patients suffering from ALS and Locked-in Syndrome.
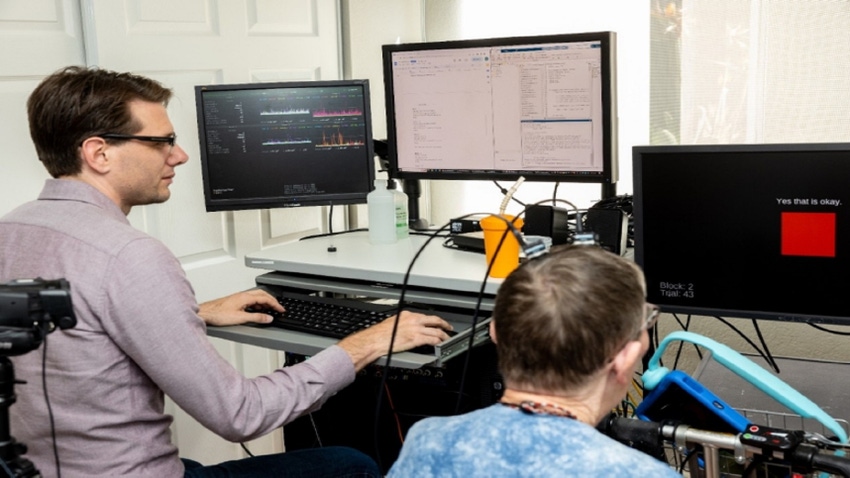
At a Glance
- New studies show AI can decode brain signals from paralyzed patients into speech and facial expressions
Researchers are pushing the boundaries of AI-powered brain-computer interface (BCI) innovations with new studies showing impressive results.
The first from Standford University, saw researchers place four tiny sensors, each the size of a baby aspirin, into the brain of a patient suffering from amyotrophic lateral sclerosis (ALS), a neurodegenerative disease that targets neurons that affect movement, which can eventually result in paralysis.
Two pairs of sensors were surgically placed in two separate brain regions that affected speech production. The specially-designed components are square arrays of 64 electrodes that penetrate the cerebral cortex. The arrays were attached to thin gold wires that exited through pedestals attached to the skull and connected to a computer with a cable. The system is part of an intracortical brain-computer interface (iBCI).
Software was then used to decode neural activity to demonstrate speech-to-text BCI – with AI algorithms receiving and decoding data coming from the test subject’s brain.
The software eventually taught itself to distinguish distinct brain activity used to compose each of the 39 phenomes that make up spoken English. The platform could decipher brain activity used for speech and translate the data into words on a screen.
ALS usually begins by causing weakness in the limbs, hands and digits. For Pat Bennett, ALS first attacked her brain stem, which affected the muscles of tongue, larynx, jaws and lips, impacting the way she enunciated phonemes, or units of sound, like “sh.” She was still physically mobile and could use her fingers to type. Her brain could continue to transmit signals to generate phonemes, but her mouth couldn’t verbalize the sounds.
The scientists worked with Bennett to start research sessions (twice a week for four months) to train the software to translate her speech. A recurrent neural network (RNN) decoder was used to emit the probability of each phoneme being spoken at each time. The RNN was trained on the data in combination with the previous days’ data using customized machine learning methods based on modern speech recognition. The neural data available was limited, but 10,850 sentences were collected in the training dataset by the final day. The researchers used two different language models: a large set with 125,000 words for general English usages and a small set with 50 words for expressing simple sentences.
By the end, the BCI system could express 62 words per minute, which is three times as fast as the previous attempt at BCI-assisted communication. The error rate for the large set was 23.8%, the first demonstration of large-vocabulary recording. The small set showed an error rate of 9.1%.
In another breakthrough from scientists at University of California San Francisco and UC Berkeley, a patient named Ann, suffering from locked-in syndrome (LIS), could communicate by translating her brain signals into speech and a digital avatar.
Her neural activity is synthesized into speech patterns based on a wedding speech the patient gave before she suffered from a brainstem stroke. Her facial movements were represented in a digital avatar.
The BCI system involves a paper-thin rectangle of 253 electrodes placed on the brain’s surface. The electrodes intercept the brain signals that would’ve signaled muscles to the patient’s larynx, lips, tongue and jaw. A port positioned in the patient’s head has a cable that connects the electrodes to a computer bank.
The researchers worked with Ann to train the algorithms to decipher her unique brain signals associated with speech. From a 1,024-word vocabulary set, she repeated different phrases until the software recognized the brain activity patterns associated with the basic sounds of her speech.
Speech Graphics, an AI-powered facial animation company, worked with the scientists and Ann to simulate muscle movements in a digital simulation of Ann’s face. The team developed customized machine learning processes to mesh the company’s software with signals from Ann’s brain and translate them into movements in her avatar’s face, including opening and closing her jaw and moving her tongue. The digital avatar was also able to capture facial expressions for surprise, sadness and happiness.
The platform converted her brain signals into speech and facial expressions at a rate of almost 80 words per minute. Ann’s current communication system allows for 14 words per minute. The team is hoping to develop an FDA-approved system for wider use.
About the Author(s)
You May Also Like