Analytical KM: A new approach to knowledge management
Analytical KM: A new approach to knowledge management
March 10, 2020
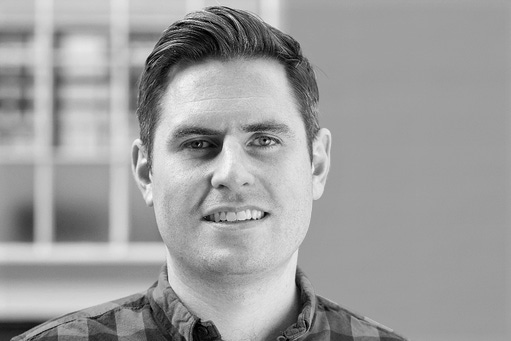
by Joe Campbell, RAVN
10 March 2020
Knowledge management (KM) has always been an essential aspect of a well-run enterprise, but there isn’t just one type. Different styles of KM – and even regional variations – have emerged over the years.
In the beginning,
there was traditional KM. This was focused on organizing knowledge
within the enterprise, mapping out processes, and documenting best
practices.
In the past decade
or so, businesses entered an “innovation era,” bringing on board
specialist teams and process improvement experts, creating innovation
hubs, and willingly embracing AI and other cutting-edge technologies.
Out of this
innovation era, we have seen the emergence of a new type of KM that
we might call analytical KM. Analytical KM taps into the data that is
naturally created as an output of these innovative processes that
have been put in place to enable data-driven decision-making.
Analytical KM in action
Consider an organization that manages a property portfolio. Traditional KM would map out the process and suggest innovative ways of executing that process, potentially using existing knowledge to remove some of the negotiations involved.
Analytical KM,
meanwhile, would use AI to review and extract data points from the
property leases to identify opportunities. If the leases are in a
high rise, and 50 of the leases are coming up for renewal in the next
3 months, the organization can take a proactive role in either
continuing or discounting those leases based on what the data is
showing. That’s the kind of data-driven decision-making analytical
KM enables.
This same
data-driven approach can be used to efficiently tackle the upcoming
LIBOR
rate change. Many organizations have hundreds – or
thousands – of contracts that are tied in some way to LIBOR. Using
AI enables these organizations to find all of the contracts that are
tied to the LIBOR rate and calculate the potential impact, so that
the organization can take appropriate next steps.
The act of
collecting know-how also reveals the difference between traditional
and analytical KM. The traditional KM approach is to collect this
know-how by asking people within the organization to state their
areas of expertise or sending out email requests for the best example
of a particular type of document. Analytical KM uses AI to
automatically surface experts in a particular specialty area or to
find the best example of a knowledge document housed within a
document management system.
Knowledge graphs are
at the heart of this analytical process. A knowledge graph is a
technology that links data together, looking at the connections
between different data points – for example, what projects a
particular person within an organization tends to spend most of their
billable time on (which can identify expertise), or how many
different people have downloaded and used a particular contract or
template (which can identify the best knowledge document to use).
If this concept
sounds familiar, it’s because companies like Netflix and Amazon use
knowledge graphs every day to curate and make recommendations based
on the connections between different pieces of data. Consider it
analytical KM on an industrial scale.
Regional differences, similar goals
In addition to these different styles of KM, there are slight regional variations. In the UK, knowledge management is dominated by creating content and know-how, whereas the United States is more technology and search focused. Put another way, the UK tends to actively document knowledge within the organization, whereas the U.S. tends to search and uncover knowledge within the organization.
In all cases,
however, the goal is the same: to actively use knowledge to improve
the way the organization conducts business. Data-driven
decision-making will become the norm over the next 3-5 years, which
means that analytical KM will only grow in importance. Embracing this
new approach to knowledge management will allow businesses of all
types to better use the data within the organization to uncover
insights that drive change, create efficiency, and identify
opportunity.
Joe Campbell is product manager for iManage RAVN, an artificial intelligence platform that powers a number of applications to automatically organize, discover and summarize documents.
About the Author(s)
You May Also Like