Google unveils DeepCTRL: A rules-focused approach to deep learning training
Training method could see use in physics and health care spaces, paper suggests
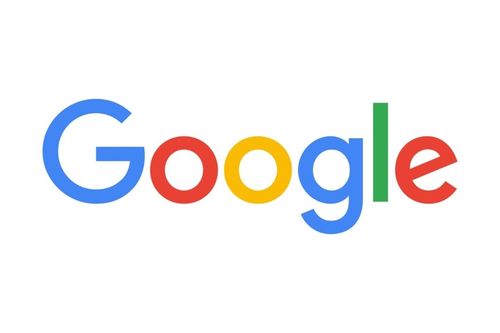
Google researchers have unveiled DeepCTRL: a new approach to deep learning training.
The system, officially titled (Deep Neural Networks with Controllable Rule Representations) incorporates a rule encoder, which the minds behind it claims can enable shared representation for decision-making.
A 16-page paper detailing the novel method suggests it does not require retraining to adapt the rule strength – at inference, as the user can adjust it based on the desired operation.
Such an advantage would “help generalize DeepCTRL to non-differentiable constraints,” a Google AI blog post by the research teams reads.
“DeepCTRL ensures that models follow rules more closely while also providing accuracy gains at downstream tasks, thus improving reliability and user trust in the trained models."
“Additionally, DeepCTRL enables novel use cases, such as hypothesis testing of the rules on data samples and unsupervised adaptation based on shared rules between datasets.”
DeepCTRL has potential use cases in physics and health care, where the researchers suggest the utilization of rules in terms of machine learning applications is “particularly important.”
Training deep learning systems from rules has “multifaceted” benefits, according to the scientists, including providing extra information for cases with minimal data, minimizing inconsistencies and reducing the impact of slight input changes.
“Learning from rules can be crucial for constructing interpretable, robust, and reliable deep neural networks,” the researchers said.
“We demonstrate three use cases of DeepCTRL: improving reliability given known principles, examining candidate rules and domain adaptation using the rule strength.”
Figure 1:
Image: DeepCTRL pairs a data encoder and rule encoder, which produce two latent representations that are coupled with corresponding objectives. The control parameter α is adjustable at inference to control the relative weight of each encoder.
About the Author(s)
You May Also Like