How machine learning can tackle insider threats
With the baseline of “normal” behavior established, a machine learning-enabled NDR solution can then extrapolate into what is unusual behavior
August 11, 2020
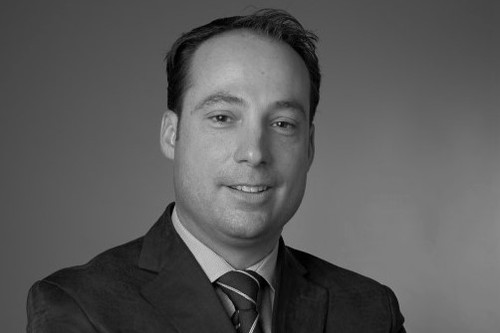
Sponsored Content
With the baseline of “normal” behavior, a machine learning-enabled NDR solution can then extrapolate into what is unusual
The question of the insider is one that has dogged enterprises, organizations and anyone with something to keep secret for millennia.
The notion that someone close to you, “someone on the inside,” is waiting to steal your secrets and compromise your systems is a risk that can never be quite banished.
And though the insider threat can be found anywhere there are secrets to be kept, the centrality of network computing to our modern way of life and the incredible complex multiplicity of data flows make the insider a particular concern for cyber security. In fact, Verizon’s 2019 Data Breach Investigations Report revealed that 30 percent of data breaches were caused by insiders.
Indeed, few moments pass by in the cyber security world without someone mentioning the ubiquitous threat of the insider. And yet, behavior often doesn’t match up with the chatter.
Cyber defense often points conspicuously outwards. A 2017 Andersen Research report showed that organizations spend 75 percent of their security budgets on prevention alone. Enterprises like to focus on perimeter defenses, but it’s what happens behind those walls that they should really care about.
Intruders have gotten very good at getting past those perimeter defenses, or otherwise circumventing them entirely. Often that involves the creation of an insider threat—that can happen in a number of ways. For high-level nation state backed groups who are looking to get into very valuable, very secure areas, a person will likely be directly recruited by bribing, blackmail or coercion. But for your run of the mill cyber criminal, the kind that most enterprises face on a daily basis, it’s a lot easier.
That could involve getting access to legitimate user credentials inside the enterprise. As recent years have shown us, it’s not too hard to find password information creeping around the web, or pluck the “pa55w0rd” style credential from a post-breach dump of information. Attackers also frequently use social engineering to get users or IT support personnel to divulge credentials—an special worry now that most people interact with IT support remotely.
From there, an attacker can leverage that user’s privileges to move laterally throughout the network. As they hunt for ever more authoritative credentials and the broad powers they provide, they get closer to critical systems and data. Many organizations are completely blind to those final stages of an attack.
By focusing on manning the walls, the keep is left largely unprotected. The problem is that the insider was already waved past the gates and is now heading towards the crown jewels and the keys to the kingdom.
The notion of post-compromise is important here. Whether the threat is coming from an adversary who has gained access to the network or an insider, you need to detect their activity to stop the attack before the network is breached. To defend against threats no matter how they found their way inside, organizations should think about enabling greater east-west visibility, but that in itself is a tough job. A Security Operations Center (SOC) gets on average a few thousand of alerts a day. And when the information is that thick, it becomes a smokescreen, obscuring more than it illuminates.
But when it comes to a cyber security expert detecting these threats, you’re not looking for their ability to gleefully rifle through traffic data, or examine meaningless alerts or carry out thousands of menial tasks. What you want is their insight and their experience. You want their strategic mind, not their brute strength.
This is where a machine learning-enabled Network Detection and Response (NDR) solution comes in. At the most basic level this potent combination learns what regular and irregular behavior looks like. By utilizing network data to observe how a system functions, how users interact and what resources they access, you can create a baseline of behavior.
Creating that baseline involves passively monitoring data from the communications of users and devices. Network observation can prove particularly useful in this case as it provides a top down, objective picture of what’s happening that can’t be evaded. A common phrase that you will hear is “packets don’t lie.” Other methods of monitoring behavior can be evaded by savvy attackers who can disable endpoint agents or delete log files.
But most importantly to stop a threat post-compromise you need to monitor both the north-south and the east-west traffic. Reliance on the ingress and egress on the perimeter traffic has led far too many to fall victim to an insider threat. To catch a threat inside your network you need to profile and look deeply into the east-west lane too—where dwell time gives adversaries the time they need to breach the network.
With the baseline of “normal” behavior established, a machine learning-enabled NDR solution can then extrapolate into what is unusual behavior—so when a user is accessing something they normally don’t, logging in from an unrecognized IP or exfiltrating a suspiciously large amount of data, it’s flagged.
Many security tools rely on rules or signatures which throw up thousands of false positives a day for what is effectively normal behavior. What might seem like an insider threat inside one organization will seem completely normal in another.
The ultimate goal of any security solution that you bring into your environment is that it should improve the lives of your boots on the ground. Your overworked analysts need access to the data that will help them investigate events and know when to escalate incidents. They need to make faster decisions with a guide to help them through the steps they need to take by laying out the breadcrumbs to follow. Ultimately analyst efficiency and productivity will ensure that your teams are more satisfied in their roles and that your risk is lowered. By freeing your human experts from the menial labor of wading through alerts and providing the intelligence they need at their fingertips—an NDR solution can improve your security posture.
There’s much that machine learning is touted to offer to many different disciplines, but amid its myriad of promises, cyber security experts should look very closely at what it can offer their teams. Insiders might always be a risk, but the combination of machine learning and network data means that you can sleep better at night knowing that you have the data you need to stop a compromise before it inflicts damage on your organization.
Mike Campfield is VP, GM, International and Global Security Programs at ExtraHop.
You May Also Like