Upping the Ante: Transmuting Machine Learning into Verifiable Knowledge
Upping the Ante: Transmuting Machine Learning into Verifiable Knowledge
August 16, 2018
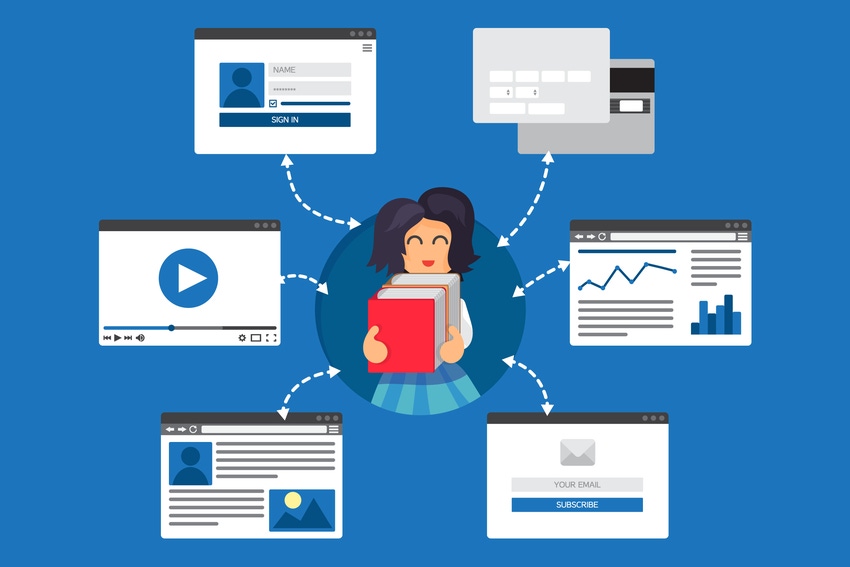
By Jelani Harper
Machine learning is arguably at the forefront of contemporary artificial intelligence technologies; many widely (if not mistakenly) consider the two terms synonymous. But in their zeal to capitalize on this latest wave of data-driven technologies, a number of organizations have struggled with ways to transfer machine learning results into tangible business value.
Common machine learning use cases include automation capabilities for expediting aspects of data engineering, customer micro-segmentation for targeted marketing or sales, and decision support systems for informed action in an array of industries. In most of these instances, machine learning results are used semi-autonomously for individual purposes, and not readily contextualized with the rest of the data available to the enterprise.
However, there’s a growing number of use cases indicating organizations can maximize machine learning’s ROI by actually inputting the results of its intelligent algorithms back into the databases providing their initial information, creating an assortment of highly nuanced query results based on what is now verifiable AI knowledge. According to Franz CEO Jans Aasman, these machine learning deployments not only maximize organizational investments in them by driving business value, but also optimize the most prominent aspects of the data systems supporting them.
“You start with the raw data…do analytics on it, get interesting results, then you put the results of the machine learning back in the database, and suddenly you have a far more powerful database,” Aasman said.
Machine Learning Concepts For Actionable Knowledge
The principal distinction between this use of machine learning and conventional isolated uses is the former benefits the enterprise and its IT systems as a whole, whereas the latter avails the individual leveraging it. It’s the difference between individual and communal knowledge, engendering the greatest impact for machine learning with a recurring value that’s practically limitless.
For internal applications, organizations can use machine learning concepts (such as co-occurrence—how often defined concepts occur together) alongside other analytics to monitor employee behavior, efficiency, and success with customers or certain types of customers. Aasman mentioned a project management use case for a consultancy company in which these analytics were used to "compute for every person, or every combination of persons, whether or not the project was successful: meaning, done on time to the satisfaction of the customer."
Organizations can use whichever metrics are relevant for their businesses to qualify success. This approach is useful for determining a numerical rating for employees “and you could put that rating back in the database,” Aasman said. “Now you can do a follow up query where you say how much money did I make on the top 10 successful people; how much money did I lose on the top 10 people I don’t make a profit on.”
Once those query results are input, organizations can keep issuing queries and inputting their findings for more specific queries predicated on the knowledge provided by previous results. The output of these analytics are an excellent means of determining which employees are most suitable for particular customers or business opportunities, as well as which aspects of their behaviors they need to improve to heighten productivity.
Related: Streamlining Data Science and Analytics Workflows for Maximum ROI
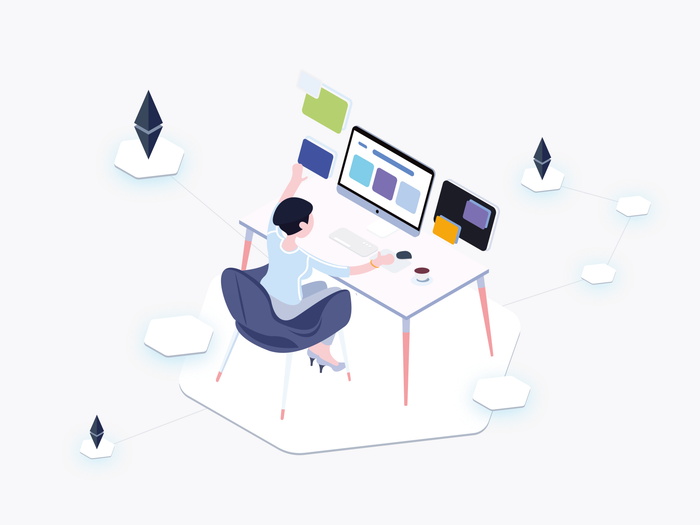
Cognitive Probability
The crux of this advanced analytics deployment is inputting machine learning findings back into databases. Although there are several means of doing so, one of the most readily available—especially for harmonizing data of varying structures and sources—is to use a knowledge graph approach in which machine learning results are converted to triples and reinserted into their graphs. There are astounding examples of this method’s potential in the life sciences and healthcare spaces, particularly for uncannily accurate predictions related to patient health, pharmaceutical development, and even genetics.
Organizations with large enough datasets can determine the probability of the effects of certain chemicals and compounds in pharmaceuticals, for example, to decrease time to market and costs associated with research and development. In healthcare, inserting the results of machine learning back into big data systems can effectively unveil “a person’s medical history” Aasman indicated. “In the future we expect that a doctor will have a typical system where they see your medical history, but with this you can see your medical future. It will say details of your genetic information and all the other information you can think of. You can kind of see the predictions of what you’re going to get, or what you should test for or [how to] take preventative action.”
Aasman has been involved in such an undertaking for several years with his efforts in the creation of the Semantic Data Lake for Healthcare in conjunction with Montefiore Health System. With access to approximately 3 million patient records spanning roughly 10 years, the data lake can be used to compute the co-occurrence between medical conditions in patients, so practitioners can discern the likelihood of patients developing additional conditions based on those they already have.
Inputting those findings back into the database can grant manifold options related to healthcare since, for instance, if cluster analytics were used to determine the likelihood of future patient events “now being in a cluster is part of the definition of a patient,” Aasman explained. “Now the next data scientist can say, ‘give me all the people from cluster one, and look at how in this cluster, this diagnostics relates to that diagnostics’."
Better Analytics, Better Data
The practice espoused by Aasman of inserting machine learning results back into databases inherently increases the worth of the analytics performed, and of the databases housing the data deployed for queries. Consequently, users effectively “build on top of previous analytics,” Aasman mentioned. The possibilities of this process are virtually interminable.
On the one hand, they’re responsible for increasing the specificity and accuracy of analytics results. On the other, “if you get better analytics for the same thing, then we can just delete the old analytics and put new analytics in there,” Aasman commented. “We can compare the analytics we did in 2016 with the analytics we did in 2017 to see if anything changed in that year.”
Jelani Harper is an editorial consultant servicing the information technology market, specializing in data-driven applications focused on semantic technologies, data governance and analytics.
About the Author(s)
You May Also Like