Why deep learning will be the most transformative technology of 2020
Why deep learning will be the most transformative technology of 2020
January 15, 2020
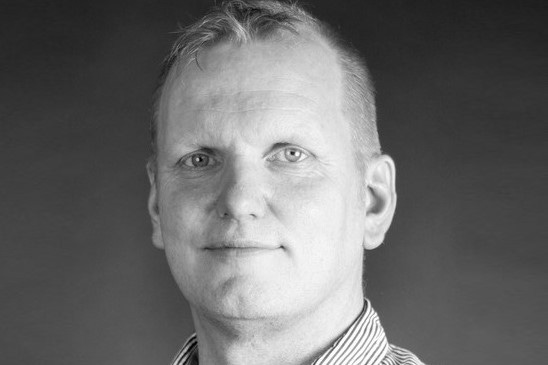
by Björn Brinne, Peltarion
15 January 2020
The AI sector is booming; with spending set to almost triple by 2023 and advancements like the NLP model BERT about to transform the way AI understands text, new use cases are emerging every day.
As AI continues to gather pace in the year ahead, organizations need to act now to ensure they do not get left behind – with this in mind, here are the top four AI trends to keep an eye on in 2020.
It’s time to get deep
One of the interesting trends in AI is the growing popularity of deep learning; a subset of machine learning (ML) that uses neural networks. In fact, a recent report from Coleman Parkes found up to 99% of AI decision makers plan to invest part of their R&D budgets on deep learning initiatives over the next three years. Typically, most other types of machine learning require a significant amount of input from data scientists on the algorithm itself before it can start solving problems. For example, for most machine learning algorithms to recognize a cat, they will need to be programmed to recognize the different characteristics of a cat – something that can be rather hard to explain and do. With deep learning, you can instead just show multiple images of cats and the model will start to know a cat by sight, which is much easier and more accurate. As such, deep learning is more adept at “unsupervised learning,” drawing insights from otherwise unusable data that may be completely unstructured or unlabeled. Simply put, deep learning is machine learning at its most powerful.
This ability to solve complex problems faster, and to analyze images by sight instead of description, has several useful applications – whether it’s making quick and accurate diagnoses in healthcare using scans and health readings, using audio sensors to identify machines that require maintenance or providing deeper personalization for retail firms.
However, aside from a handful of top firms, deep learning has traditionally been too difficult for most companies to deploy, but this is all set to change in 2020. As a recent study from Peltarion and CognitionX explained, increased data availability, developments in processing hardware and improved neural network models are making it easier to access deep learning. In other words, we’re finally ready to start deploying deep learning effectively in business environments. Next year, organizations will start using deep learning to their advantage, reaping the benefits and using it to help achieve their goals.
It’s good to talk
Within the field of deep learning, new transformer-based NLP models such as BERT (Bidirectional Encoder Representations from Transformers) and XLM-R are set to open up a wide range of use cases for virtually any organization. Historically, deep learning has primarily been used for image processing, which has seen it used most commonly in healthcare. The technology has been particularly successful in healthcare for analyzing CT scans, for example, where it can quickly and accurately identify cancerous areas that can be targeted for radiotherapy treatment. However, over the past year, these new methods have been successful at solving complex problems using unstructured text data to a very high standard.
Suddenly, deep learning models are available that will understand the semantic meaning of the text it reads, going beyond the basics. This creates a lot more opportunities for deep learning to be used more widely. These applications include everything from dealing with customer inquiries in the contact center or monitoring social media sentiment, to helping financial services firms looking to support decisions with more accurate market predictions, or even deciphering legal contracts or invoices.
2020 will see the race to “explainable AI” intensify
A recent report from consultancy firm OC&C found that a key challenge of AI adoption is building trust in the answers it produces – organizations need to know how a model came up with an answer and prediction to ensure that these decisions are not tainted or biased. For many regulated industries, such as finance and healthcare, this is essential; imagine going to a bank and being refused a mortgage with no explanation except ‘computer says no’. So, a big trend we are seeing now is a scramble to create a more transparent and ‘explainable’ AI that can give us these answers. Even defense organizations, like DARPA in the US, are looking into ways to solve this issue.
This is a trend we are seeing several of the big companies, and specialist companies like us, working on presently – in fact, we have just started an Industrial PhD program to focus solely on explainable AI over the next four years.
AI will become increasingly internationalized, helping to unlock deep learning’s potential
We are seeing faster progress than ever in AI; discovering the best ways to develop deep learning techniques using powerful resources, identify new use cases using NLP and work on explainable solutions that are ready for business use. However, in order to move forward, we need to focus on making connections to real-life problems and delivering value for companies. The potential value of deep learning is immense, so it’s vital that it becomes more widely usable across companies of all sizes.
We are on a good path to fix this, however, as new operational AI tools are being made available that will allow more people and businesses to work with neural networks, not just academic researchers. By taking a platform approach to AI, organizations can help reduce the resource requirements to use deep learning, making it more scalable and affordable. What’s more, making deep learning more widely usable will increase the speed of progress and create more business use cases to help drive uptake.
Björn Brinne is Chief Data Scientist at Peltarion. Björn has been at the head of AI projects for major organizations like King (developers of Candy Crush) as well as other gaming firms such as Electronic Arts. Now, he works with operational AI firm Peltarion, using his skills to help companies solve real world problems with deep learning in areas such as finance, healthcare and manufacturing.
About the Author(s)
You May Also Like