Exclusive: Dell EMEA's CTO on AI and Multicloud
Elliott Young joins the AI Business podcast to explain how generative AI fits in with a company's multicloud strategy
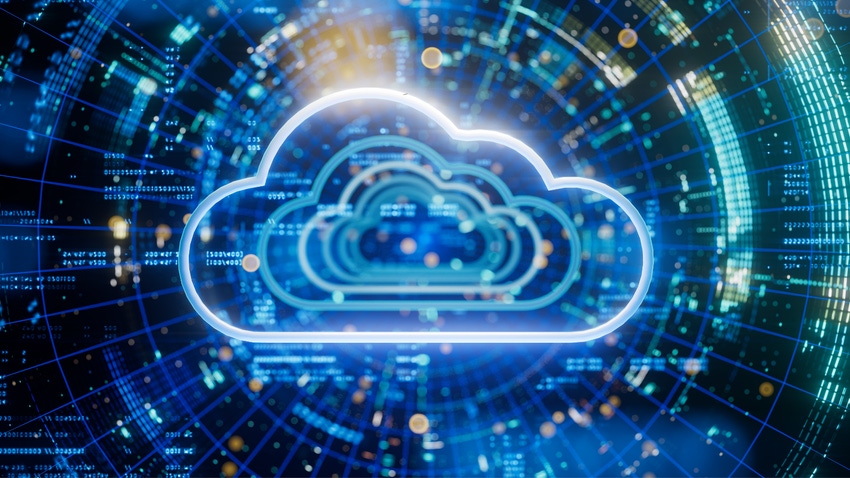
Elliott Young, CTO of Dell Technologies EMEA, joins the AI Business podcast to discuss AI and multicloud. He outlines ways in which businesses should consider adopting a multicloud approach to deploying generative AI models.
Listen to the podcast below or read the edited transcript.
Give us a brief overview of your role at Dell.
At Dell, I am the CTO for the core division within Europe, Middle East and Africa. In practice, that means forming bridges and close relationships with board-level customers. We are looking for ways to optimize their existing IT and look forward to what kind of strategy they need to constantly evolve and adapt to take advantage of new technologies.
Can you outline for our audience what a multicloud approach is and how it relates to AI?
There are a couple of different ways to look at this. Multicloud starts by thinking about what kind of operations or IT approaches do you have on-premises and what you want to leverage in a multicloud, or in a partner's cloud. We call that ground to cloud – it is the idea that you can host an AI-optimized workload on the solution, like PowerFlex, for example, and have that same capability running on-premises or in Azure or AWS, and have seamless access to data in a simple way with one control plane to manage it all.
The second consideration is whether there are capabilities that you want to have in a public cloud, which you can then bring to a different location, which could be on-premises or to a partner site. We look at what we can do to optimize the usage of data between multiple clouds. You may have a federated approach where as soon as you write the data into one cloud, like Azure, it instantly pops up in AWS. And then you can access that data in any way you want to without the overhead of copying it between clouds or suddenly getting a huge charge from your cloud provider because you moved some data from one place to the other. These are the kinds of things that we are looking at and asking, ‘What is the optimum solution?’ And surprisingly, it changes quite frequently.
What are some common use cases you see for applying AI to multicloud - whether it is cost optimization, security, workload placement, etc.?
It depends on what kind of AI we are talking about. Companies have been doing machine learning for several years. But are they doing that on-premises or in a public cloud? If we take the example of machine learning, there is a clear use case to separate training in a machine learning environment to inferencing.
A typical design pattern that I see is where organizations want to do their training somewhere close to where the data is to get a kind of data gravity effect. But then they might take that data, put it in an inferencing container and place it closer to whatever is going to consume the output from machine learning. I might take the container and run it in Azure, or I might take the container and run it in an Edge Gateway in a factory somewhere. I think that is a great use case for thinking about how to separate the different components from machine learning.
Generative AI infrastructure is very different from typical machine learning infrastructure. Here you are making conscious decisions on where is the best use of things like GPU cycles or CPU cycles. With machine learning, typically you will have a catalog of capabilities or models and you can run those either on CPUs or GPUs. With generative AI, it is more common to have a GPU-accelerated approach, so you have got to think about costs and flexibility. These are some of the considerations that people are thinking about with different types of AI.
What about security considerations? Does that differ over multiple types of cloud you are using?
Let us take those two examples again − machine learning versus generative AI.
With machine learning, if you want to do your training in a public cloud or using one of the various ‘as-a-service’ offerings, you have to consider questions like how am I going to anonymize the data before I put it into that cloud service? How am I going to change it to reference keys as opposed to actual financial transactions? You also have considerations around copying in terms of performance, risk and security. And then when it gets to that final destination, you have suddenly invalidated the opportunity to do compression or DeeJoop, because you have to use whatever that provider is hosting for you.
On the generative AI side, one thing that people are finding as they are implementing is that, if you are not careful, it is easy to create an escalation of privilege or to bypass things like role-based access control. That is because it is so easy to hook up a large language model to your own data via an index or a vector database, that as soon as the AI has got access to it, all of a sudden, it is an expert on everything that is in that dataset. And then you start thinking about, ‘Do I want the cloud service provider to also maybe have access to that in some way?’
What issues are clients coming to you saying they’d benefit from a multicloud environment to improve their AI?
There are various techniques you can use for that. One of the considerations is, what is the tradeoff between how much work you want to do to refactor your existing setup and your existing data, versus the time to value to get something out of your new generative AI solution. Some companies start by saying, ‘We need to fix our data or change our metadata, or have better master data management.’ My view is that if companies have been working on that for many years, or even a decade and they still have not fixed their data issues, then is that realistically going to get done in the next six months so that you can use generative AI? Probably not.
The solution to think about is that unlike machine learning, generative AI is incredibly tolerant of imprecise data or data that may not be perfect. I would start by implementing a large language model platform in the most appropriate place, whether it is on-premises or in a public cloud, and then start to see what your users will do with it. And that tier zero approach is just giving them access to that kind of capability.
Afterwards, you might want to optimize your infrastructure to take in new datasets or join them together. Companies are now looking at data lakehouses – where as soon as you put the data on there, it is immediately converted to a format or capability where you can query it at the same time as you are loading it. But you can also make it available directly to any large language model that might need to take advantage of that. The design patterns for generative AI are going to have to keep up with modern trends.
Has it been tough for customers looking to change their infrastructure as generative AI emerges?
We had a customer who came into one of our executive briefing centers who had spent the last two years tuning their multicloud approach and the business came along and asked for generative AI to be included. You spent all this time architecting for one thing, and suddenly, this huge new requirement just came out of nowhere.
The surprising thing for us looking out at how our customers are using AI is that somehow the businesses, when they saw the potential of this kind of technology, new budget emerged from functional departments, as opposed to just going to the CIO asking for money. Now there is suddenly this additional pot of capability where the business units are saying, ‘You must consume this because we need to benefit from generative AI in the next few months.’
What are you talking to clients about when it comes to taking a multicloud approach to AI whilst avoiding bias and ensuring fairness, transparency and responsibility?
That is a real consideration. If you are using a public service, something similar to ChatGPT, then considerations like bias or explainability are not easily under your control. You do not have access to inspect the data used to train the model. There is a trade-off in terms of how you consume these things versus what else you can do with these models. But if you are using a cloud large language model as a kind of endpoint, you can intercept the responses from the cloud, and take some action based on the contents of that response for actions like bias detection.
There are some other interesting use cases. You might want to deploy the Falcon model. You can download the Falcon model from Hugging Face and install it on a Dell server and get it running by the end of the day. Now, in that particular case, that is quite interesting from a point of view of bias and observability because the people that created Falcon released a good chunk of the data that they trained the model on. This allows you to provide samples of what the model was trained in to answer queries about outputs. Multicloud by design approach comes into its own when you start thinking about these kinds of concepts, like bias, or transparency.
What about regulatory concerns? What are you telling customers in terms of adhering to regulations?
Just last year, we had the AI Safety Summit. And it was incredible to see people from so many different parts of the world all in one place seeming to agree that regulation is required, particularly looking at things like frontier AI. We then had, in December, the European Parliament come up with a proposed new regulation. In that regulation, they published a list of banned applications, which means that you actually will be breaking that law, once it is implemented, if you have used generative AI to implement things like emotion recognition in a workplace or a school, or if the AI is thought to be manipulating human behavior to circumvent their free will.
The nature of the relationship between computers and humans is changing. Previously, computers were just doing rudimentary things that were instructed by humans, and now it is changing to a point where it could be possible for a computer to impact somebody's rights. And the fines for that are pretty significant, so these kinds of considerations are very real.
Looking ahead, what excites you around AI and multicloud? What prospects are you most looking forward to in terms of seeing how this could help accelerate development of applications?
I am in a pretty fortunate position because I get to see all the latest and greatest technology before a lot of other people. Generative AI is mind boggling, some of the proofs of concept and demos we have done for our customers even made me stop in my tracks.
One of the things that stands out for me is when multiple AIs are working together as a team. There are solutions like AutoGen from Microsoft and ChatGPT, with OpenAI having implemented functions and plugins. When the AI gets to a certain state, it realizes it has this capability where it can make a call outside to go and look up today's weather, or a CRM record from your database and give that answer back to the human who asked the question.
That is the kind of thing that I am looking forward to seeing in the future, because you can take these different components and build them across multicloud solutions. That little function that did the callout into your CRM database, that function was probably running on premises while the large language model could have been in the public cloud. You have got this concept of how AI is embedded into products, and then how you are picking the right part of the multicloud solution to host it.
What can we expect from Dell with regard to AI and multicloud?
Dell is all about choice. We like to give multiple different ways to achieve similar outcomes and let the customer choose what is the most appropriate for them. We have a close partnership with Nvidia. But in the same way, we have a close relationship with Intel and AMD. This gives you fantastic access to even more GPU memory which you might need if you are deploying the biggest models with the most parameters. We are working hard to make sure that if you are looking to host a particular technical solution you can always get the right infrastructure that is the right match for that solution from Dell.
Read more about:
ChatGPT / Generative AIAbout the Author(s)
You May Also Like