AI and Ethics: Risks and dilemmas
AI ethics is essential from a moral perspective, for the corporate culture, for the organization’s reputation, and from a legal perspective
January 13, 2022
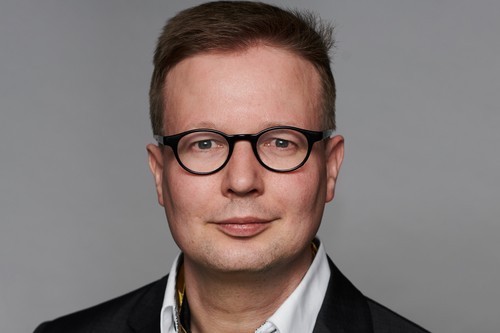
AI ethics is essential from a moral perspective, for the corporate culture, for the organization’s reputation, and from a legal perspective
Suppose your banker tells you their AI calculated a 75% chance that you get divorced within the next two years and, thus, you do not get a mortgage. Would you consider this prediction to be ethically and morally adequate?
Having worked in the Swiss banking sector for more than a decade, this is the big pink elephant in the room no banker talks about – especially not with customers. I am not aware of any bank calculating this risk.
However, the rumors indicate that this is the most significant risk for mortgage issues.
Nevertheless, making such predictions would contradict banks’ aim with most other companies, organizations, and corporations: being perceived as good citizens. They pledge to higher standards than “Don’t be evil.” Such aims or pledges have implications for their analytics and AI initiatives. The initiatives improve revenues and customer satisfaction while reducing costs.
However, suppose customers, society, politicians, and prosecutors perceive an AI project as unethical. In that case, AI becomes a reputational or even a legal burden. How can managers and AI project leaders prevent that?
The three areas of ethical risks
Ethical risks for AI fall into three categories, as Figure 1 illustrates:
Unethical use cases
Unethical engineering practices
Unethical models
Figure 1: A lifecycle perspective on ethical questions in AI
Unethical use cases such as calculating divorce probabilities are not AI topics. They are business strategy decisions. Data scientists can and should require a clear judgment on whether a use case is acceptable or not. This judgment is with the business, not with the IT or AI side of an organization.
Ethics in engineering relates to the development and maintenance process. For example, Apple contractors listened to and transcribed Siri users’ conversations enabling Apple to improve Siris’s AI capabilities. This engineering and product improvement approach resulted in a public outcry since it was seen as an invasion of the users’ privacy [Rob20].
Finally, the ethical questions related to the AI model and its implications for customers, society, and humans are the true core of any AI ethics discourse. When algorithms decide who gets the first Covid vaccination [Wig20, Har20] and governments outsource the AI model development to Palantir [Fie20], AI ethics is not an academic question. It decides about life and death.
AI and ethical dilemmas
The discourse about ethics and the AI model has two aspects: model properties and decisions in ethically challenging situations or ethical dilemmas, for which no undisputable choices exist.
The classic example is the trolley problem, which also applies to autonomous cars or assistant systems for drivers in a car. Ahead of a trolley are three persons on the track. They cannot move, and the trolley cannot stop. There is only one way to stop the trolley from killing these three persons: diverting the trolley. As a result, the trolley would not kill the persons on the track. It would kill another person that would not get harmed otherwise. What is the most ethical choice?
The trolley problem pattern is behind many ethical dilemmas, including autonomous cars deciding whether to hit pedestrians or crash into another vehicle.
When AI directly impacts people’s lives, it is mandatory to understand how the AI component chooses an action in challenging situations. In general, there are two approaches: top-down and bottom-up. In a bottom-up approach, the AI system learns by observation.
However, ethical dilemmas are pretty seldom. It can take years or decades until a driver faces such a dilemma – if at all. Observing the crowd instead of working with selected training drivers is an option to get training data quicker. The disadvantage is that the crowd also teaches bad habits such as speeding. [EE17]
The alternative is the top-down approach with ethical guidelines helping in critical situations. Kant’s categorical imperative or Asimov’s Three Laws of Robotics are examples. [EE17] Asimove’s rules or laws are [Asi50]:
A robot may not injure a human being or allow a human being to come to harm.
A robot must obey orders given to it by human beings except where such orders would conflict with the First Law.
A robot must protect its own existence as long as such protection does not conflict with the First or Second Law.
Kant and Asimov are intriguing but abstract. They require a translation to be applicable for deciding whether a car hits a pedestrian, a cyclist, or a truck when an accident is inevitable.
In practice, laws and regulations clearly define acceptable and unacceptable actions for many circumstances. If not, it can be pretty tricky.
Following a utilitarian ethic – looking at the impact on all affected persons together – requires quantifying suffering and harm [EE17]. Is the life of a 5-year-old boy more important or the life of a 40-year-old mother with three teenage daughters?
An AI model making an explicit decision whom to kill is not a driver in a stressful situation with an impulse to save his own life. It is an emotionless, calculating, and rational AI component that decides and kills – and here, society’s expectations are higher than for a human driver [YSh18].
What complicates matters are contradicting expectations. In general, society expects autonomous cars to minimize the overall harm to humans. There is just one exception: vehicle owners want to be protected by their vehicle, independent of their general love for utilitarian ethics when discussing cars in general. [EE17]
The implementation of top-down ethics requires close collaboration of data scientists or engineers with ethics specialists.
Data scientists build frameworks that capture the world, control devices or combine ethical judgments with the AI component’s original aim. It is up to the ethics specialists to decide how to quantify the severity of harm and make it comparable – or how to choose an option if there are only bad ones.
Ethical aspects of AI models
Ethical dilemmas in AI get broad public and academic interest. However, companies and organizations have a more hands-on approach. They want to know how to create ethically and morally adequate AI models, which decide, for example, which passengers are subject to more thorough airport security checks.
Well known in this area is the early work of Google’s former AI ethics star Timit Gebru. She pointed out that IBM’s and Microsoft’s face detection models work well for white men but are inaccurate for women of color [Sim20].
Missing fairness or biased data can be the root cause for such inadequate models. Suppose a widely used training set for face detection algorithms contains primarily pictures of old and young men and boys and only a few females’ images.
If training data does not reflect the overall population, it is biased. When data scientists use this training data to create models, the resulting models work better for males than females.
Fairness has a different twist. It demands that a model has a similar quality level for all relevant subgroups independent of their actual share of the population [CR20]. Suppose a company has 95% female employees. In that case, a face-matching algorithm working well only for females can have outstanding overall results and be the best possible solution.
However, suppose it performs really poorly for the small male employee minority and does not detect anyone. In that case, society considers the model to be ethically questionable. So, fairness means to balance the model quality for subgroups, even if harming the overall accuracy.
Figure 2: Bias & Fairness. On the left, the training data does not represent the population’s actual distribution (bias). To the right, the training set reflects the population well. However, this can be an issue if, due to the small number of green persons, the model performs much worse for them than for the blue majority (missing fairness).
Governance for AI ethics – learnings from the Gebru/Google fiasco
Organizations risk emotional and bitter fights if the governance over AI ethics is not crystal-clear. The Gebru/Google controversy illustrates this.
As mentioned above, Gebru worked initially on fairness topics. After joining Google, she also co-authored a paper that criticized Google’s work on a new language model for two reasons.
First, Google trained its model with online text, which might be biased, resulting in a biased model. Second, she criticized the massive energy consumption of the language model. [Sim20]
The three areas of AI ethics in Figure 1 - Use Case, Engineering Practices, AI Model - help understanding the controversy that resulted in the termination of her contract with Google. Her research shifted away from pure ethical aspects of models such as fairness towards criticism of a language model initiative essential to Google’s future business.
Weighting business strategy and its impacts on ecology is a valid ethical question, just not at the core of AI ethics.
Organizations can learn from this controversy the need for a clear mandate. What does an AI ethics board discuss and decide? What are the competencies of corporate social responsibility or business ethics teams? What discusses and decides the top management?
AI ethics is essential from a moral perspective, for the corporate culture, for the organization’s reputation, and from a legal perspective. Thus, a frictionless integration in the company and its corporate culture is essential.
Klaus Haller is a senior IT project manager with in-depth business analysis, solution architecture, and consulting know-how. His experience covers data management, analytics and AI, information security and compliance, and test management. He enjoys applying his analytical skills and technical creativity to deliver solutions for complex projects with high levels of uncertainty. Typically, he manages projects consisting of 5-10 engineers.
Since 2005, Klaus works in IT consulting and for IT service providers, often (but not exclusively) in the financial industries in Switzerland.
[Asi50] I. Asimov: I, Robot, Gnome Press, New York City, US, 1950
[BY14] N. Bostrom, N., E. Yudkowsky: The ethics of artificial intelligence. In K. Frankish & W. Ramsey (Eds.), The Cambridge Handbook of Artificial Intelligence (pp. 316-334). Cambridge: Cambridge University Press, UK, 2014
[CR20] A. Chouldechova, A. Roth: A Snapshot of the Frontiers of Fairness in Machine Learning, Communications of the ACM, Vol. 63, No. 5, May 2020
[EE17] A. Etzioni, O. Etzioni: Incorporating Ethics into Artificial Intelligence, The Journal of Ethics, Vol. 21, 403–418 (2017)
[Fie20] H. Field: How Algorithms Are Helping to Determine Covid-19 Vaccine Rollout, Dec 21st, 2020, https://www.morningbrew.com, last retrieved Feb 13th, 2021
[Har20] D. Harwell: Algorithms are deciding who gets the first vaccines. Should we trust them?, The Washington Post, Dec 23rd, 2020
[Rob20] CJ Robbles: Apple’s Siri Continues to Listen to Conversations, Despite Last Year’s Controversy, https://www.techtimes.com, May 25th, 2020, last retrieved Feb 13th, 2021
[Wig20] K. Wiggers: COVID-19 vaccine distribution algorithms may cement health care inequalities
[YSh18] H. Yu, Z. Shen, C. Miao, C. Leung, V. R. Lesser & Q. Yang: Building Ethics into Artificial Intelligence, IJCAI’18
About the Author(s)
You May Also Like