AI and sustainable construction using ‘foamed concrete’
Study: Machine learning algorithms formulate better building materials
September 5, 2022
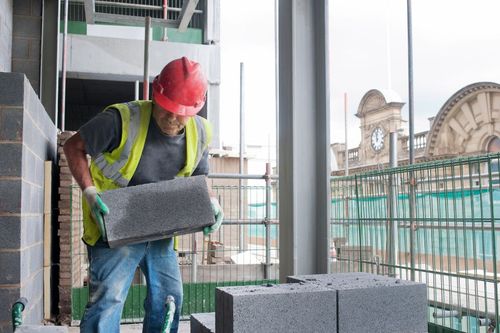
Study: Machine learning algorithms formulate better building materials
Concrete is widely used in construction, but it has a large carbon footprint and is a key contributor to climate change. There is a more sustainable replacement called ‘foamed concrete,’ but finding the optimal mixture of ingredients to make it is complex, takes time and is costly.
Now a team of scientists is applying machine learning to predict the compressive qualities of concretes before manufacture, saving time, cost and reducing waste, according to a paper published in in Cement and Concrete Composites.
Foamed concrete, also known as lightweight cellular concrete or low-density foamed concrete, is made of cement, foaming agents, aggregate, and water. Its light cellular structure uses a binding mortar to support air voids, which ultimately reduces the weight of the building materials. It is already being used in the U.K., Canada and Korea.
The material has excellent physical, mechanical, and physiochemical properties for construction. The type of foaming agent, granulometry of aggregates and cement minerology are variables that can be optimized. Water quality, mixture proportions, and the uniformity and type of pores are other important characteristics. Furthermore, the curing method plays a role in the end product.
AI-based models have the capability to optimize the mixture of foamed concrete materials for high strength-to-weight ratio for structural product performance. It can also find the best formula for energy consumption, thermal conductivity, and fire resistance.
Currently, scientists use empirical models based on complex complications to factor in characteristics, such as the relationship between compressive strength and material properties. The paper focused on using machine learning to forecast the compressive properties of concretes before production. Machine learning can help predict structural behavior.
The researchers used three machine learning algorithms with nonlinear capabilities: GBT, GEP, and ANN. The GBT model performed the best with the highest validation data. Sand to cement ratios and water to cement ratios were analyzed using parametric analysis.
The research showed a high correlation between compressive strength and foamed concrete density. The algorithms demonstrated an accurate prediction of the compressive strength of foamed concrete, proving how machine learning can accurately optimize foamed concrete for the construction industry.
The team suggested future studies could involve varying the foaming agent dosages in the machine learning algorithms to find an improved formula for foamed concrete.
You May Also Like