The key to trust is real AI governance
If organizations are to scale AI to help make business-critical decisions, they need to understand exactly how and why those decisions are being made
January 28, 2021
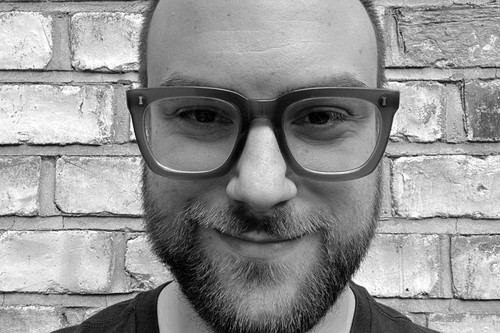
Sponsored Content
Around the world, companies are accelerating their digital transformation journeys: adopting new business models, moving workloads to the cloud and digitalising their operations.
But to truly unlock the power of their data, they are also increasingly exploring how AI can help predict and shape future outcomes, empower people to do higher-value work and streamline critical processes.
However, even as the promise of deploying AI at scale has never been greater, many organisations are left with AI models in a proof-of-concept purgatory. Why? For many, the core issue is a lack of trust in AI and its outcomes. If organisations are to scale AI to help make business-critical decisions, they need to understand exactly how and why those decisions are being made.
To harness the power of AI toward decision-making that profoundly impacts people’s lives—for example, whether a customer is approved for a home loan or a job candidate advances to the next stage—they need to be able to explain those decisions to internal stakeholders (like business owners and model validators) and external stakeholders (such as regulators).
Therefore, the key to trust is real AI governance, which requires visibility into AI models at every stage of the lifecycle: from data collection to model development; to deployment, ongoing monitoring and management.
The pillars of trusted AI
When examining the different stages of the lifecycle and the elements that form trusted AI systems, we’ve identified five pillars that resemble a holistic overview:
Fairness: AI systems should use training data and models that are free of bias, to avoid unfair treatment of certain groups.
Robustness: AI systems should be safe and secure, not vulnerable to tampering or compromising the data they are trained on.
Value alignment: AI systems should have the ability to reason through different outcomes, discriminate between ‘good’ and ‘bad’ decisions, and ensure outcomes truly wanted.
Explainability: AI systems should provide decisions or suggestions that can be understood by their users and developers.
Transparency and accountability: AI systems should include details of their development, deployment, and maintenance so they can be audited throughout their lifecycle.
Just like a physical structure, trust can’t be built on one pillar alone. If an AI system is fair but can’t resist attack, it won’t be trusted. If it’s secure but we can’t understand its output, it won’t be trusted. To build AI systems that are truly trusted, we need to strengthen all the pillars together.
Scaling AI and mitigating risks
While more and more organisations scale their use of AI, they’re challenged with mitigating the associated risks and building genuine trust in AI decision-making. When it comes to trustworthy AI, we believe that consumers, clients, and all stakeholders need to know how AI impacts their day-to-day lives, organisations, and work.
A recent Morning Consult survey of AI professionals commissioned by IBM, analysed whether trust is a barrier to AI adoption. The survey found that 84% of AI professionals agree that consumers are more likely to choose services from a company that offers transparency and an ethical framework on how its data and AI models are built, managed and used.
These findings further emphasise the importance organisations are placing on trusted AI and transparency, so much so, they believe it would impact their likelihood of gaining new customers.
In order for their AI services to be trusted, enterprises are now faced with addressing the issues of vulnerabilities, such as exposure to bias, lack of explainability, and susceptibility to adversarial attacks.
Building trustworthy AI
Assembling documentation about an AI model’s important features, such as its purpose, performance, datasets, characteristics, and more can help drive trust in the technology.
It’s been recognised that fairness, robustness, value alignment, safety, explainability, transparency and accountability are all critical to trustworthy AI. Yet, to achieve trust in AI, making progress on these issues will not be enough; it must be accompanied with the ability to measure and communicate the performance levels of a system on each of these dimensions.
One way to accomplish this would be to provide such information via factsheets for AI services. Like nutrition labels for foods or information sheets for appliances, factsheets for AI services would provide information about the product’s important characteristics, helping as organisations streamline their compliance and reporting processes, furthering efforts to build consumer and enterprise trust in AI services.
Sample questions from a factsheet might include:
Does the dataset used to train the service have a datasheet or data statement?
Was the dataset and model checked for biases? If “yes” describe bias policies that were checked, bias checking methods, and results.
Was any bias mitigation performed on the dataset? If “yes” describe the mitigation method.
Are algorithm outputs explainable/interpretable? If yes, explain how is explainability achieved (e.g. directly explainable algorithm, local explainability, explanations via examples).
Holistic approach to AI is vital for trust
Trustworthy AI is about having a holistic approach to AI governance that brings together tools, solutions, practices, and people to govern AI responsibly across its lifecycle. By implementing solutions such as an AI factsheet, organisations can better measure and communicate the products important characteristics, in turn helping to mitigate the associated risks when scaling AI.
And so, as enterprises move from experimenting with AI to widespread adoption, at scale, AI model lifecycle management and automation is quickly becoming the next frontier in AI development and research.
As Partner and AI Practice Leader at IBM UK Global Business Services, Michael helps to shape strategy from his proven expertise embedding complex AI solutions at scale. Michael is an experienced delivery leader and product owner who currently leads some of our largest engagements in the AI domain. He can often be seen speaking at industry conferences on AI in Banking, an industry in which he has particularly deep expertise.
You May Also Like