What is Responsible AI?
Learn about responsible AI, the scope of using AI responsibly, and what the regulatory framework may look like going forward.
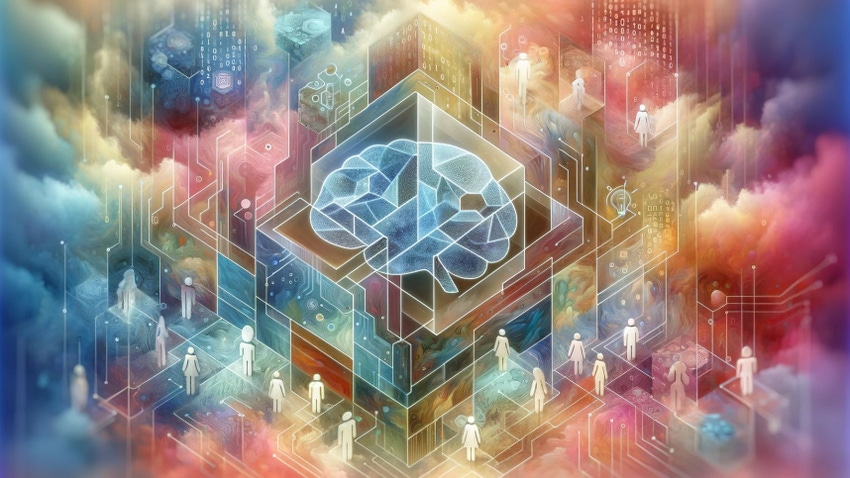
Using AI responsibly has become a key topic, as AI takes center stage in the world’s discourse. Optimism for the technology is often met with calls for caution, as well-known shortfalls become more apparent with growing usage.
Responsible AI is a way of developing and deploying AI in an ethical and legal way.
Governments around the world are keeping a close eye on AI, readying themselves to regulate once the technology has matured.
However, the vacuum left by the as yet lack of clear regulation and understanding of the technology has put the onus on tech companies to consider these ethical applications.
That means each business must do its part by embedding responsible AI practices and a robust AI compliance framework into the company. This includes controls for assessing the potential risk of AI use cases at the design stage and a means to embed responsible AI approaches across the business.
The broad nature of the technology presents a problem in terms of regulation and protecting end users from harm. It is also posing a challenge for regulators to predict when a particular outcome of an AI service will be malicious. This has resulted in increasing amount of interest from governments and regulators around the world, which have been accelerating efforts to assess what level of regulatory involvement is required.
Initiatives to regulate the use of AI are becoming more important as AI continues to advance at an unprecedented pace, especially generative AI. As with other new and emerging technologies, many regulators are cautious about over-regulating the AI sector before it has fully matured, as this could stifle innovation. However, it is equally important that consumers are protected as the technology develops. This could involve governments and policymakers amending existing policies and regulatory frameworks to reflect technological developments or setting entirely new frameworks. Generally, some form of consensus is beginning to appear regarding the need to regulate high-risk situations, for example in health care settings.
The importance of Responsible AI (RAI)
When used incorrectly, AI has the potential for discrimination and bias at industrial scale. The central problem with black box AI is that statistical methods such as neural networks are largely inexplicable to anyone but data scientists, meaning the decisions they automate are not transparent to the wider population. This makes it impossible for the reasoning that lies behind these decisions to be examined. Machine learning operates by seeking patterns in data, rather than following clear rules of logical inference as humans do. As a result, they can easily draw irrational conclusions from unbalanced data, and it can be difficult for humans to understand why, certainly on a case-by-case basis.
While there is a case to be made against consciously biased algorithms, many biased AI decisions stem directly from the datasets the algorithms have been fed. If too many white male faces are fed into a facial recognition algorithm at the expense of other demographics, for example, then the algorithm learns to associate “faces” with “whiteness” or “maleness.”
Getting AI right for public use then means getting the data right. The overwhelmingly male-dominated nature of the field, coupled with institutional recruiting bias and the lack of a supportive environment for women and ethnic minorities in the field, can correlate directly with incidences of bias in AI decision-making.
For these reasons, it is important that responsible AI approaches are implemented by companies both using and creating AI.
Best practices for implementing Responsible AI (RAI)
The changing nature of the applicative context, the possible imbalance in available data causing bias, and the need to back up the results with explanations, are adding an additional trust complexity for AI usage. There are several crucial elements to overcome these challenges and build AI responsibly.
1.Merging domain knowledge with RAI expertise
AI experts and data scientists are often at the forefront of ethical decision-making – detecting bias, building feedback loops, running anomaly detection to avoid data poisoning – in applications that may have far reaching consequences for humans. They should not be left alone in this critical endeavour.
To select a valuable use case, choose and clean the data, test the model, and control its behaviour, you will need both data scientists and domain experts.
For example, take the task of predicting the weekly HVAC (Heating, Ventilation, and Air Conditioning) energy consumption of an office building. The combined expertise of data scientists and field experts enables the selection of key features in designing relevant algorithms, such as the impact of outside temperatures on different days of the week (a cold Sunday has a different effect than a cold Monday). This approach ensures a more accurate forecasting model and provides explanations for consumption patterns.
Therefore, if unusual conditions occur, user-validated suggestions for relearning can be incorporated to improve system behaviour and avoid models biased with overrepresented data. Domain experts’ input is key for explainability and bias avoidance.
2.Anticipating risks in RAI
Most of current AI regulation has to do with applying a risk-based approach, for good reason. AI projects need strong risk management, and anticipating risk must start at the design phase. This involves predicting different issues that can occur due to erroneous or unusual data, cyberattacks, etc., and theorizing their potential consequences.
This enables practitioners to implement additional actions to mitigate such risks, like improving the data sets used for training the AI model, detecting data drifts (unusual data evolutions at run time), implementing guardrails for the AI, and, crucially, ensuring a human user is in the loop whenever confidence in the result falls below a given threshold.
3.Building RAI usage guidelines for employees
To ensure trust in AI systems, organizations must define ethical principles for AI development and build an effective governance structure around them that is led from the top.
It is also important to introduce policies and guidelines for use of generative AI applications by employees. These policies need to capture not only the use of proprietary business AI tools, but also potential use of third-party AI applications by employees using company data. In ddition to such policies, we have introduced training across the organization to ensure all employees understand the implications of using company data with generative AI applications.
Another core component of the AI governance strategy is to ensure it does not breach key compliance and privacy requirements by feeding sensitive data into external APIs provided by major AI platforms. This is particularly important in health care where the integrity of patient data is critical, so any AI use that can pose even the slightest risk to patient data needs to be prevented.
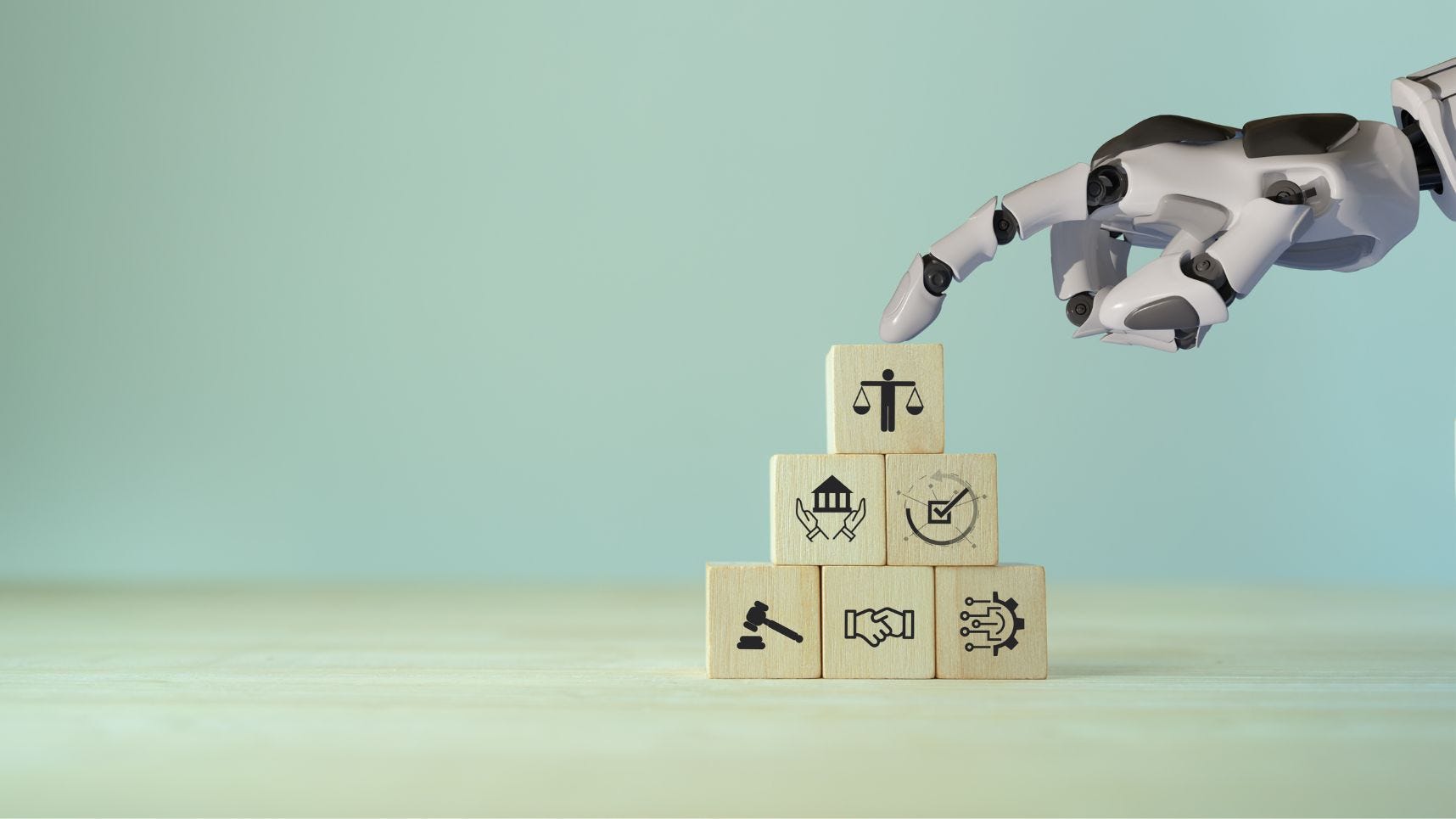
Getty images
4.Creating RAI attributes for guideline development
For example, if you’re creating a computer vision model that’s answering a straight-forward question like “is this a human?” you need to actually define what you mean by “human.” Do cartoons count? What about court sketches? What if the person is partially occluded? Should a torso count as “human” for your model? What about just a hand? This all matters. You need clarity on what “human” means for this model. If you’re unsure, just ask people the same question about your data. You might be surprised by the ambiguities present and the assumptions you made going in.
At this point, you should know both what you’re solving for and what could go wrong. In essence, you should know “what is this thing we’re building?” and “what are some things that might go wrong for our end user?” Once you have a framework here, it’s of paramount importance to deeply review your data.
After all, this is where bias is often hidden. A few years back, researchers at the University of Washington and the University of Maryland found that doing an image search for certain jobs revealed serious underrepresentation and bias in results. Search “nurse,” for example, and you’d see only women. Search “CEO” and it’s all men. The search results were accurate in certain ways – the pictures were indeed of nurses and CEOs – but they painted a world in which those jobs were uniformly held by women or men, respectively. This is just one example, but it shows how bias can lurk in data without you being able to readily identify it.
You need to think about these issues when you’re reviewing your data. It’s one of the reasons why having a diverse team involved is crucial. Diverse backgrounds help ensure that your team will be asking different questions, thinking about different end users, and, hopefully, creating a technology with some empathy in mind.
5.Better data generation for improved language models in RAI
After all, machine learning models learn from data. Good data makes good models, bad data makes bad models, and biased data makes biased models. In fact, the steps customers take to tune models to remove bias is directly analogous to how a customer tunes a model to account for changing business conditions or algorithmic uncertainty, generally. It all boils down to getting better data.
Be transparent and open regarding what data trained the system, where it was collected, how it was labeled, what the benchmark for accuracy was, and how that’s measured. Declare the purpose of the decision-making and the criteria through which that decision is made. Be empathetic. Understand that you will have different end users and they’ll all use your system differently. Imagine what their experiences might be and build for those, in addition to the ones you inherently expect.
Take feedback! Ensure there is a mechanism to request question and answer, get a human judgement, or gracefully fall-back in low-confidence situations to not be overly-reliant on an AI system. Just like humans, it’s okay for the robot to say, “I’m not sure.” Learn! When an outcome is questioned, ensure there is a way to give feedback, retrain, and ensure that the model is actively learning from new examples and real-world data.
It’s not impossible to reduce unwanted bias in your models. It takes some grit and hard work, to be sure, but it reduces down to being empathetic, iterating throughout the model building and tuning processes, and taking great care with your data.
Regulatory challenges of AI in relevance to RAI
AI has its own fair share of debate, except that it seems the voices of these groups are louder, and concerns are far greater, than for other emerging technologies. Approving the adoption of a certain technology just because it has gained adequate support, or discarding it due to a fear of opposition, is not considered good practice for policymakers. It is the duty of the government and policymakers to diligently weigh up the risks and benefits of the technology before arriving at an opinion.
It is important that regulators strike the right balance when it comes to regulation, as there are consequences for the market of under- or over-regulation. There are several challenges facing regulators when developing responsible AI regulation:
Ensuring safety standards for RAI
Safety is the most basic feature that any government considers when approving the deployment of not just AI, but any machinery or technology that directly or indirectly possesses a threat to life or property, or that could cause economic or financial losses. The threat is exponentially higher in the case of AI due to its ability to act independently and with minimal human interference.
Imposing safety standards on AI software is just as important as imposing them on AI agents. Policymakers will need to take special interest in framing safety standards in areas that directly or indirectly involve a greater threat to life or property from deployment of AI, such as aviation, transportation, mining, logistics, and defence. In the near future, it would not be surprising if governments set up a safety board or agency and obligated companies to get safety approval from these authorized entities to permit the deployment of AI.
RAI and Its Role in Privacy, Data Management, and Copyright
AI decisions are based on the underlying data and its algorithms, which are designed to evolve over time with experience. The greater the amount of data, the easier it will be for the AI to make meaningful patterns and emulate human behaviour. However, gaining access to useful data has raised privacy concerns across the world, with most countries already rolling out data protection laws such as the General Data Protection Regulation (GDPR).
Furthermore, provisions in data protection laws that mandate data controllers and data processors to disclose the purpose of data collection and its usage will pose a big challenge, as this cannot be clearly defined while it is being fed to AI applications. The gradual adoption of AI will eventually force governments and policymakers to revisit data protection measures to make amendments to the existing data protection laws, to ensure a trade-off between protecting users’ privacy and data collection.
Recent innovations in AI, such as generative AI, are raising new questions about how copyright law principles such as authorship, infringement, and fair use will apply to content created or used by AI systems. AI programs are trained to generate outputs by exposing them to large quantities of existing works, and this calls into question whether the systems might infringe copyrights. Not only this, but the use of generative AI programs also raises the question of who holds the copyright to content created using these programs — the user, the program, or the program creator/owner?
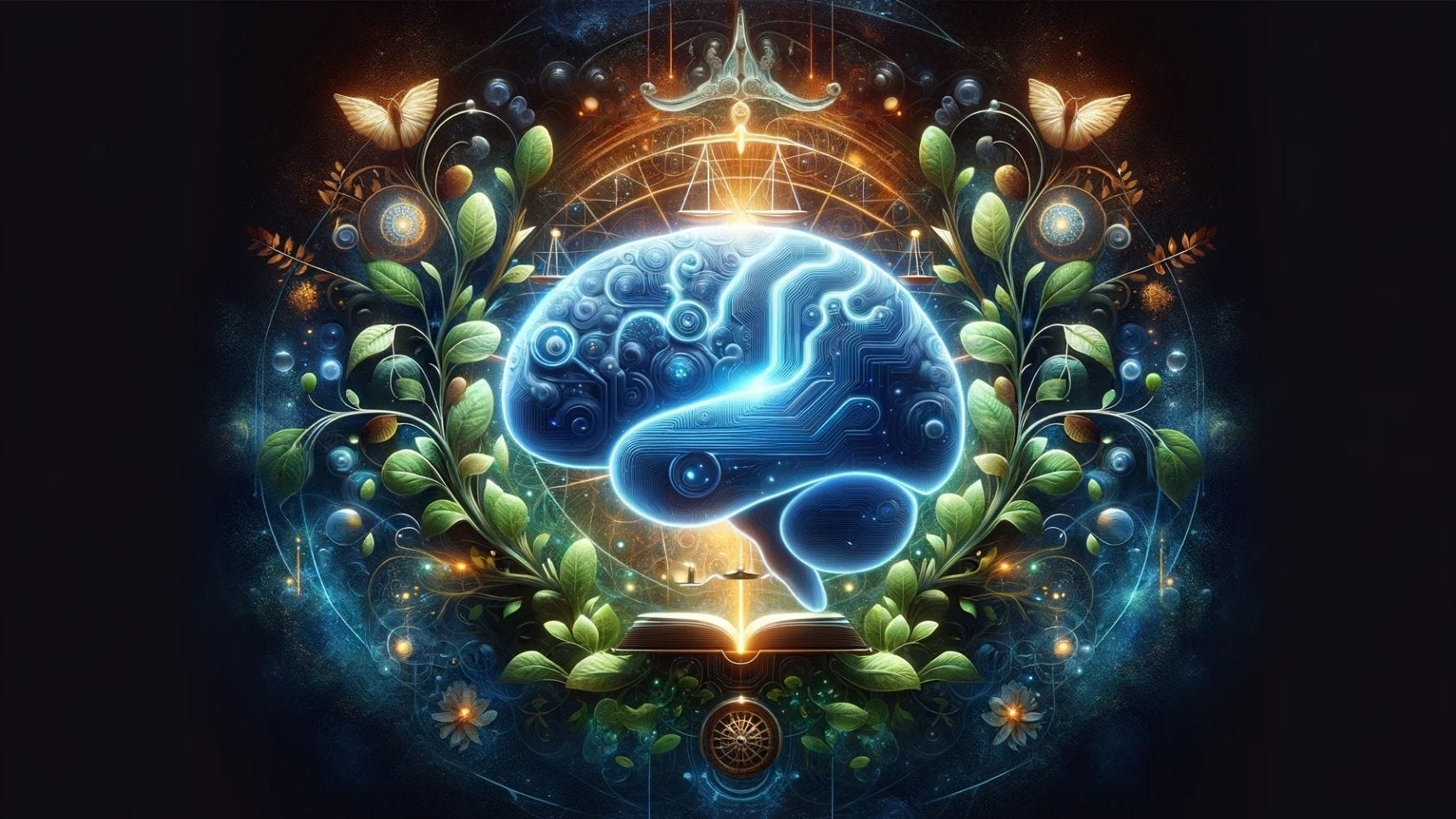
AI Business via DALL-E 3
Ensuring AI's Controllability
The current developments and deployments of AI are still in the early stages. While a few AI systems are fully autonomous, the majority are still supervised and controlled using human interference. In time, and with further advancements, the human supervision and interference will reduce, paving the way for a greater number of AI applications that are completely autonomous. However, the failure of such systems due to external factors such as exposure to unfamiliar scenarios, a breach in security, or damage to components and sensors, or internal factors such as software malfunctions and inefficiently trained systems, would result in greater damage, especially across industries that involve critical decision-making, such as aviation, automotive, defence, and health care.
The policymakers should enforce a controllability code within AI applications that will enable humans to take control of the system during a time of uncertainty. Furthermore, the controllability will enable human interference in cases where the AI system deviates from the intended duties it was designed to perform, or when it identifies that a certain task is outside of its limits. In parallel, efforts on controlling the data fed in to train the AI system will help to develop a trustworthy system.
Upholding Ethics in AI
Ethics play the most pivotal role in shaping regulatory policies on AI, with several governments across the world already incorporating an ethics code into their national strategies. Because the development and training of an AI system is a continuous process that involves developing algorithms and feeding in data, as well as monitoring and updating the system, policymakers should be extra cautious about defining the ethical guidelines. The guidelines should help avoid unfair bias, prevent the input of inappropriate data to train AI systems, maintain respect for privacy, and avoid development of AI systems with an undisclosed agenda on which the very fundamentals of the AI applications would be built.
Developing a trustworthy and robust AI system will involve multiple professional stakeholders, such as developers, statisticians, academics, and data cleansers. Policymakers and governments should work alongside each other to invest in and train professionals to incorporate the best ethical practices into their AI systems. Arguably, this is not something new to governments, as many already allocate a considerable amount of funds to training citizens to improve digital skills as part of their respective national plans. In the future, AI companies are expected to choose to introduce a new AI ethics officer role or board to monitor and safeguard ethical values incorporated into AI systems, similar to the role of a data protection officer.
Advocating transparency and accountability in RAI usage
For a system to be trustworthy, transparency plays a crucial role, and with the involvement of AI systems that not only collect data but also make decisions, AI companies must be even more cautious in gaining the trust of consumers, even in sectors where there are no stringent regulatory measures. Therefore, policy designers must ensure that, as far as possible, AI companies are transparent, both fundamentally and technically.
Fundamentally, the regulator should mandate that an AI system must disclose its identity beforehand and should empower users with the right to reject the interaction and obligate provisions through other modes of communication. The regulator should also obligate AI systems to disclose information about any data they intend to collect and divulge the purpose of this data collection to users in the interest of users’ privacy, especially in scenarios where the users are under AI surveillance.
Technically, policymakers should make provisions in the regulations to obligate AI companies to make their systems as transparent as possible; these include algorithms and any reasoning behind why and how the AI system has arrived at a certain decision, especially when it is created for public use. However, policymakers need to strike a balance between maintaining transparency and corporate interests. This is because imposing heavy transparency obligations could create challenges for AI companies when it comes to protecting their intellectual property and could deter progress in the sector.
Enhancing AI’s Security
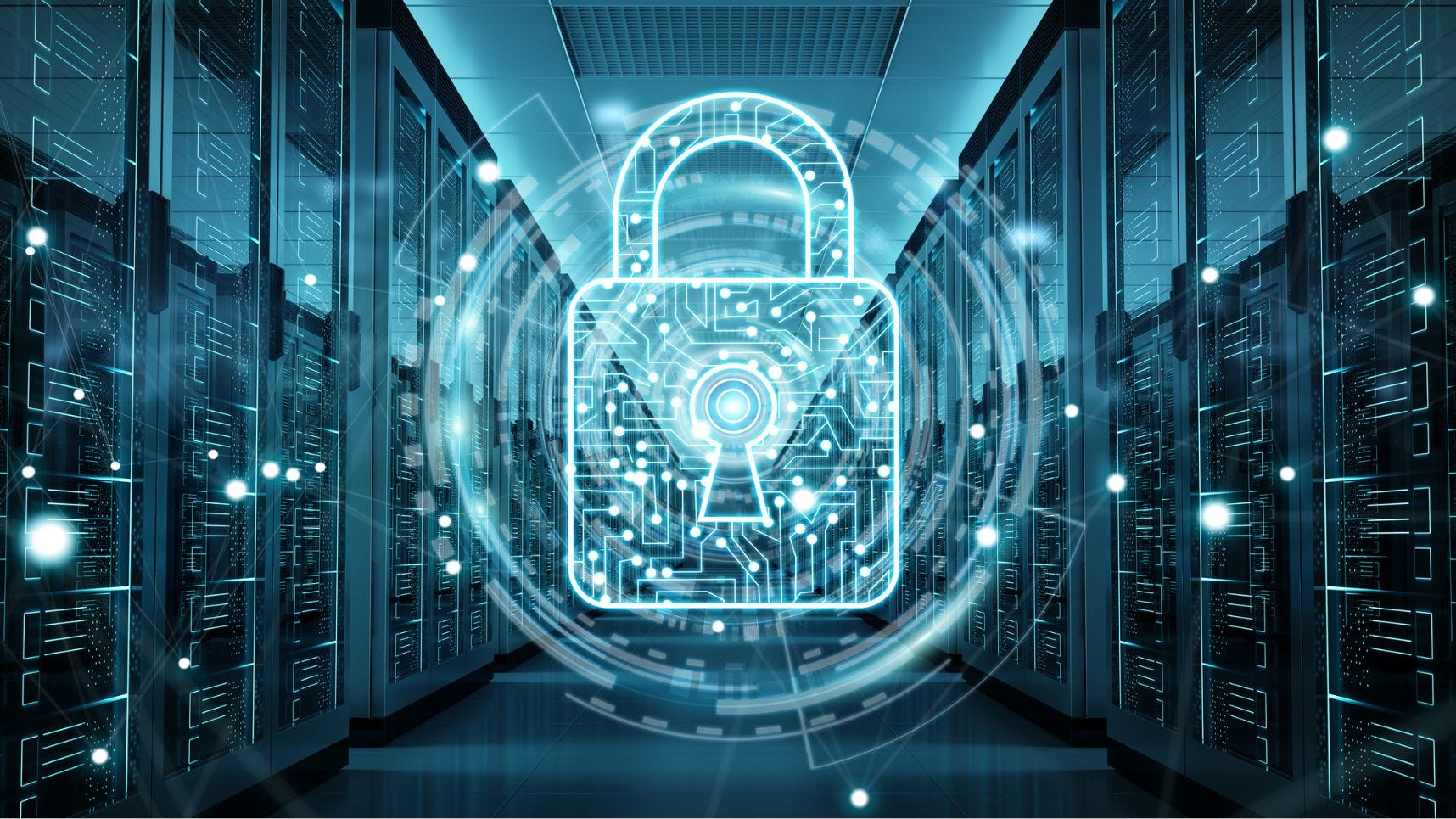
Getty Images
AI will thrive only when the users are confident that their information is secure, and that the systems are not easily vulnerable to threats. The threat is even more sophisticated if the data that is fed into the AI system for learning is hacked, and the AI system is misled. For AI systems to be secure, the companies should ensure not just physical security but also security of the system across the entire value chain, from networks and data to software. Although building a secured AI system is more of a concern to the company developing it than just the regulators, policymakers should look to set technical standards, such as encryption standards for storing data and cybersecurity controls to prevent unauthorized access.
Furthermore, policymakers can define certification standards that the companies need to fulfil to ensure that the AI system is secure, both physically and internally, for commercial deployments. These can cover the following:
Robustness
Respective industry board standards
Technical standards (more so when the AI system is for public use)
Over the period of AI system deployment growth across industries, policymakers can introduce more refined industry-specific standards to gain the trust of users.
Promoting collaboration and interoperability across the AI ecosystem
The development and adoption of an AI ecosystem is still in a nascent stage. For society to embrace new technology, there must be a collaborative approach between various stakeholders, including private sector, government, academics, and citizens. Governments and policymakers together should foster the development of the necessary infrastructure and establish common platforms to share research and data to develop inclusive AI models that address the various needs of both private and public entities. Also, governments should create a roadmap to train and educate citizens to embrace the adoption of novel technologies such as AI.
Furthermore, with the AI ecosystem still in the early stages of development, to avoid dominance of a particular entity, which would set off antitrust concerns, policymakers should focus on establishing standard, internationally accepted protocols. An open interface should be created to ensure there is fair practice regarding interoperability between AI systems that should be followed by all developers and entities. Also, the rise of AI systems will pave the way for new business models and increase the number of applications for new patents. To support these changing needs, respective government departments and policymakers should set policies and licensing terms collaboratively to ensure a smooth transition.
How to regulate AI
Developing a Framework for RAI Orientation
So far, specific regulations covering the use of AI systems have not been adopted by any country. The regulatory agendas of most countries have focused on addressing certain ethical issues raised by the use of AI. These ethical issues primarily pertain to a violation of fundamental human rights, privacy, and algorithmic bias.
To address these concerns and ensure trustworthiness in AI systems, several countries have developed their own set of AI ethical guidelines or principles. For example, the EU’s ethical framework for AI is based on seven principles: human agency and oversight; technical robustness and safety; privacy and data governance; transparency; diversity, non-discrimination, and fairness; societal and environmental well-being; and accountability. Meanwhile, China provides a detailed list of principles for three key areas, including research and development (R&D), governance, and use of AI. The principles of human-centric AI in Japan focus on education/literacy, privacy protection, ensuring security, fair competition, fairness, accountability, and transparency.
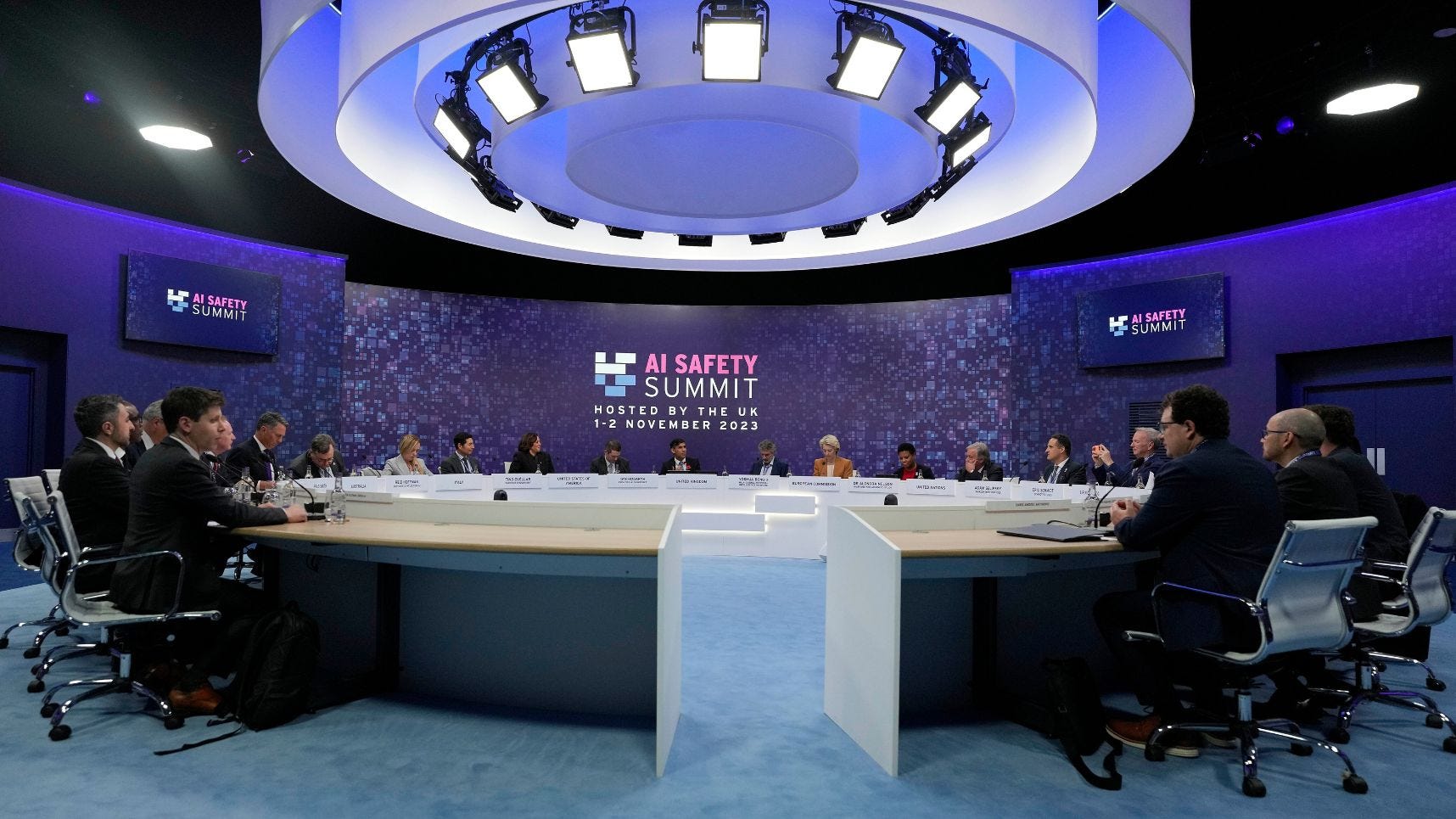
Responsible AI was at the forefront of discussions at the first ever AI Safety Summit. Credit: Alastair Grant - WPA Pool/Getty Images
To facilitate the development of AI ethical guidelines, countries have been setting up AI ethics forums and councils, which are primarily responsible for monitoring the use and development of AI technologies across sectors and providing recommendations on ethical issues. These guidelines, in most cases, are non-binding for AI developers and solution providers. To drive adoption of these guidelines, countries can consider introducing a reward and labelling mechanism, under which companies that meet the requirements of trustworthy AI would be awarded a seal or label—for example, a seal for cybersecurity and data ethics, which companies can use to brand themselves on their compliance with IT security and data ethics.
Establishing RAI observatories and knowledge centers for AI policy
Some countries are setting up AI observatories and knowledge centers, which act as a collaborative platform for all stakeholders in the AI space. The key objectives of these regulatory centers are to share insights, analyze best practices for shaping AI-related policy, and identify legal barriers of AI adoption.
Evaluating the existing legal frameworks for commercial AI use
Many governments have highlighted the need to evaluate the current legal frameworks in their national AI strategies. Outdated regulations should be revisited, especially in the area of data protection and user consent, to avoid stifling innovation and ensure the smooth integration of AI. Major concerns around collecting and safeguarding data are already addressed under existing data protection laws in respective countries, e.g., the GDPR in EU Member States. However, it is important to check if these laws need to be amended to accommodate AI, particularly to improve transparency and help governments to hold AI companies accountable. Several countries are also considering amending sector-specific regulations for AI.
Deploying AI: Setting up regulatory sandboxes
It is worth considering establishing controlled environments for AI experimentation through regulatory sandboxes. The key objective of sandboxes is to facilitate the testing of AI solutions in real-life conditions by temporarily reducing regulatory burdens.
Great power, greater responsibility
It’s right to be optimistic about AI’s future. Organizations that use it are more collaborative, evidence-based and make more informed, accurate and successful decisions. It’s not hard to see why AI is becoming a dominant trend in many sectors. Yet we cannot be blind to the risks or the need to get the basics of data governance right. AI offers great power, and with great power comes even greater responsibility.
About the Author(s)
You May Also Like