Will AI have a snowball effect on gender bias?
Will AI have a snowball effect on gender bias?
January 14, 2020
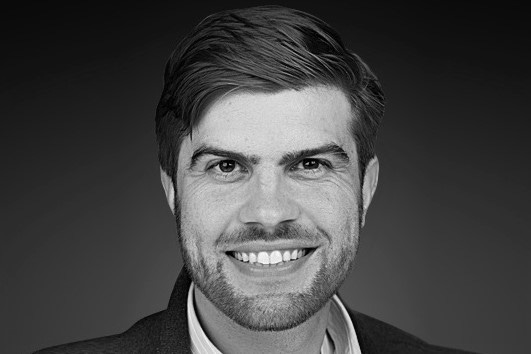
by Zachary Jarvinen, OpenText
14 January 2020
Artificial intelligence may still be a relatively young field, but some fear that it is already falling victim to the lack of diversity seen in the wider tech industry.
With LinkedIn data revealing that 78 percent of the individuals currently working in AI are men, many fear that the technology will be built by men for men, with AI tools (consciously or unconsciously) becoming biased against women – a risk for any group that has been historically under-represented in the workplace.
Industry experts are comparing the transformative potential of AI with other “general purpose technologies” such as the steam engine or electricity. As we move into an era in which business functions rely more and more on machine-enabled decision making, potential gender bias is a very real concern which organizations must confront proactively – with workplace technology likely to reflect those that populate and drive it. Datasets can be skewed, for example, and if gender stereotypes are present, machine learning models could actually amplify them. So, how can we stop AI from having a snowball effect on historical and existing workplace gender bias?
Mitigating the risk of data-driven bias
AI algorithms and systems “learn” by processing historical data, meaning that any data filled with gender stereotypes could perpetuate gender bias. Take Amazon’s AI recruitment tool as an example: trained to vet applicants by observing patterns in résumés submitted to the company over a 10-year period, the AI-driven tool was able to give job candidates scores ranging from one to five stars. Yet, because most applications were submitted by men during this time period, the data had a preferential recruitment of males and even downgraded resumes which contained the word “women”.
More
recently, the Apple
credit card ran into major problems
when users noticed that it seemed to offer smaller lines of credit to
women than to men. Goldman Sachs, the issuing bank for the Apple
Card, insisted that the
algorithm
doesn’t consider gender as an input for the application process.
However, many have highlighted that a gender-blind algorithm could
actually end up biased against women if it’s drawing on data that
happens to correlate with gender. With these recent examples of
gender bias still fresh in our mind, questions have been raised
around whether the technology be controlled to avoid unintended or
adverse outcomes?
The best way to prevent bias in AI systems is to implement ethical code at the data collection phase, beginning with a large enough sample of data to yield trustworthy insights and minimize subjectivity. Thus, a robust system capable of collecting and processing the richest and most complex sets of information, including both structured data and unstructured, including textual content, is necessary to generate the most accurate and impartial insights.
These measures to ensure data quality can never fully safeguard AI models and systems entirely against bias. It’s therefore critical that results are examined for signs of prejudices after the fact as well. Any noteworthy correlations among gender – as well as race, sexuality, age, religion and similar factors – should be investigated. If a bias is detected, mitigation strategies such as adjustments of sample distributions can be implemented. Organizations should also consider having a HR or ethics specialist collaborating with, and conducting regular check-ins and audits with, its data scientists to ensure that models and systems align with organisational values.
Striving for bias-free AI
Demand for AI skills has tripled over the past three years, although industry demand currently exceeds supply. It’s therefore vitally important that organizations do not lose the opportunities or market share that women represent. After all, AI requires a certain amount of human judgement which places more value on skills such as problem-solving, empathy, negotiation and persuasion – skills which have historically been aligned more closely with women than men.
Yet,
if we want AI systems to reflect equity, we must ensure the people
and teams building systems and adding information are just as
equitable. Research
shows
that groups that are more cognitively diverse tend to make better
decisions. In the context of AI, diverse teams with a rich blend of
views, backgrounds and characteristics are more likely to flag
problems that could have a negative social consequence before a
product or system has been launched. The issue of diversity was
raised earlier this year by the Confederation of British Industry
(CBI), who highlighted that businesses need diverse teams in place to
ensure AI does not “entrench existing unfairness”.
Looking
ahead
Companies are betting on AI because of its potential to let computers make decisions and take action. Yet, a recent survey of US and UK-based IT decision-makers revealed that nearly half (42%) are “very” or “extremely” concerned about AI bias, with many fearing that it could comprise brand reputation and, ultimately, lead to a loss of customer trust if it’s found to be present within their systems.
To mitigate the risk of unintentionally biased AI models – as well as subsequent issues this could cause for the business – the first hurdle is to ensure that datasets are free of historical prejudices. Data scientists will need to use the richest and most complex sets of information, including structured data and unstructured data, to generate trustworthy insights and minimize subjectivity.
The next hurdle is to ensure that diverse teams, with a variety of views, backgrounds and characteristics, work closely with a HR or ethics specialist to ensure that AI models are thoroughly checked for evidence of bias or discrimination. This collaboration will be essential to build equitable AI systems and to ensure the long-term well-being of the AI sector, which is certainly achievable for those who put their good intentions into practice.
Zachary Jarvinen is head of product marketing, AI and Analytics at OpenText. Prior to this, he ran marketing at for a Data Analytics company that reached #87 on the Inc 5000, was a part of the Obama Digital Team in 2008, and is a polyglot with an MBA/MSc from UCLA and the London School of Economics.
About the Author(s)
You May Also Like