The natural union of statistical AI and bots
The natural union of statistical AI and bots
February 19, 2020
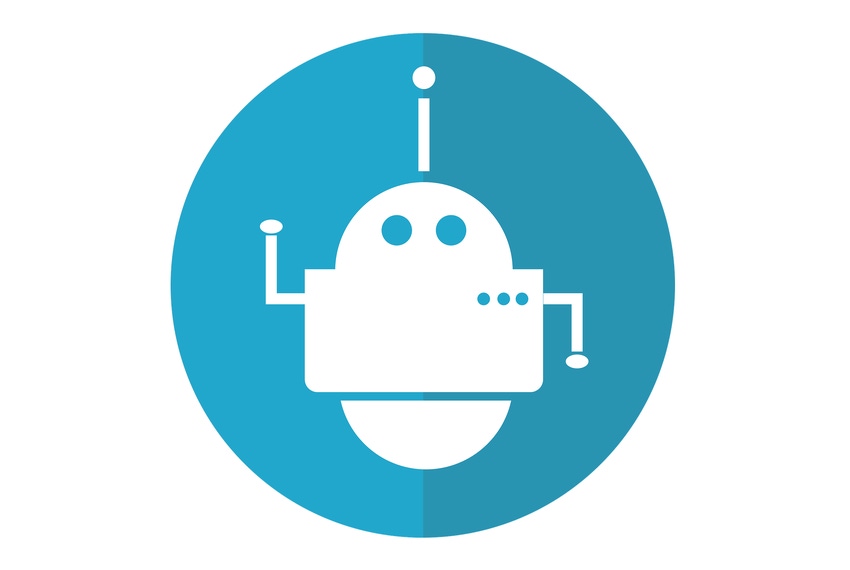
by Jelani Harper 19 February 2020
The advantages of deploying statistical expressions of Artificial Intelligence—typified by machine learning—alongside bots are abundantly clear. The former is responsible for imbuing the latter with heightened intelligence for front-end applications. Bots, in turn, create action from advanced pattern recognition that makes machine learning commercially viable to organizations.
The winner of this combination is ultimately the enterprise which, in addition to accessing machine learning, is able to benefit from automation. “What you realize is, there’s a lot that goes into [deep learning] to try to actually use it in production within the enterprise,” reflected Tom Wilde, CEO of Indico, which partnered with Cognizant to solve this problem. “Bots encapsulate the complexity to make it actually usable.”
By pairing these two technologies together, organizations can solve an array of business problems; best of all, they can do so at the scale and speed required of modern data management demands.
Implementing machine learning
Traditional approaches to shifting machine learning models from the data science sandboxes to production environments are computationally demanding and time-consuming. In-house implementations of machine learning begin with building and training models, which require extensive data engineering. Next, costly data scientists are tasked with having to “figure out what computing infrastructure you’re going to use to deploy it on, and scale it, and measure it,” Wilde explained. Once models are assessed, organizations must refine them and redo much of this work.
When leveraging statistical AI models attended by bots from progressive vendors in this space, “those requirements are encapsulated inside the bot in a manner that the customer doesn’t have to think about them so hard,” Wilde said. “Many of those steps are under the hood, if you want to think of it that way.”
Although organizations are still responsible for tailoring models to their own particular use cases, they can focus on assessing the performance of the intelligent bot in production without bootstrapping many of the logistics required for its operation. Data scientists aren’t necessary, and organizations can solve common document-based or text analytics problems without “needing very specific skills to write rules, to write software,” Wilde said. “You now only need to be able to figure out how to put enough examples in front of a deep learning algorithm in a careful enough manner to teach it how to do what you’re trying to do.”
Intelligent process automation
The array of use cases for deploying deep neural networks in the manner specified by Wilde are vast and include aspects of computer vision, the Intelligent Internet of Things, and other examples of what he termed “fuzzy problems.” Unlike straightforward ones involving structured data, these sophisticated tasks encompass “problems with not necessarily a simple right or wrong answer, but a fuzzy computing requirement to be able to learn and apply that learning onto a problem,” Wilde said. The growing assortment of unstructured data, especially in verticals such as financial services or insurance for document processing, exemplifies this reality—and is one of the areas in which the tandem of bots and statistical AI are producing measurable results.
In these industries, documents must be processed, filed, and accounted for over extremely lengthy periods of time; Wilde referenced a life insurance use case in which “those policies might sit around for decades.” These documents become the basis for rendering adjudication, loan approvals, and other important decisions in which “humans are really good at…and machines have been historically bad at,” he said. “Machines haven’t been good at the judgment component. And with the arrival of deep learning and… bots, suddenly the machine is beginning to be good at judgment and doing it at scale and at speed.”
Workflow management
The benefits of scale and speed are obvious - by deploying these technologies to process more mortgage applications quicker, organizations can also process them at enterprise scale. Similarly, by incorporating an array of unstructured data—including images, public data sources, and internal sources—to determine whether an insurance claim is approved or denied, organizations can greatly expand the scale at which they process these use cases, resulting in obvious speed advantages.
Overall, the impact of synthesizing statistical AI with bots is considerable. Bots enable organizations to simplify the logistics of using some of the most advanced approaches for machine learning. “It’s almost like a lego block,” Wilde said. “You can give the enterprise this basket of Lego blocks that encapsulate this complexity that allows them to snap them together in a way that, if they want to build a castle, or a car, or whatever that might be.”
The rapid inclusion of AI into core tasks such as implementing decisions in insurance and finance broadens the worth of this technology to the enterprise. Not long ago, it was only used in fledgling use cases in binary, deterministic (right or wrong) judgements. Today, its applications have substantially expanded to include uses that are “fundamentally probabilistic in nature,” Wilde remarked. “I think that’s the right way to think about them. The answer is a probabilistic answer, not a deterministic answer.”
Jelani Harper is an editorial consultant servicing the information technology market, specializing in data-driven applications focused on semantic technologies, data governance and analytics.
About the Author(s)
You May Also Like