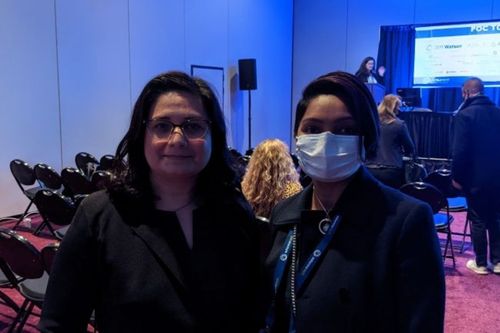
Longevity and ROI from AI can be achieved, but only with the right preparation, auditing protocols and agile development processes
Companies building artificial intelligence models are seeking longevity from their investment. But it’s harder to keep algorithms relevant when the data it ingests is changing and its operations are scaled three or five years down the line.
Saira Kazmi, executive director for engineering (data and analytics) at CVS Health, said auditing accountability was crucial to building a model that meets company objectives and should be in place from the earliest stages of development, checking off several key tasks such as explainability, bias, security and data integrity.
During the experimentation and design phase, the key is to make sure the algorithm meets a number of objectives – understanding its impact and setting out expectations for deployment, and building awareness of the underlying data science and how it relates to the business.
An end-to-end AI model life cycle includes business, engineering and data science teams, Kazmi added, and representatives of all three disciplines should be involved from the project’s outset.
“It’s about setting the expectations and thinking about how to enable the literacy of the product,” Kazmi said. “Because once your algorithms are deployed in production – say a classification algorithm with five buckets for an approval process for a job – it’s important for a [hiring manager] to know why [a candidate] was bucketed in a certain way.”
Before the project begins, enterprises must also think about how to capture, mature, access and integrate data – given the means of holding data will vary from enterprise-to-enterprise. Agile features for model implementation – for instance feedback loops and feature tracking tools – could also be considered, Kazmi added.
About the Author(s)
You May Also Like