Avoiding AI Failures: Best Practices for Implementation
An opinion piece by the manager of innovation at Alcatel-Lucent Enterprise
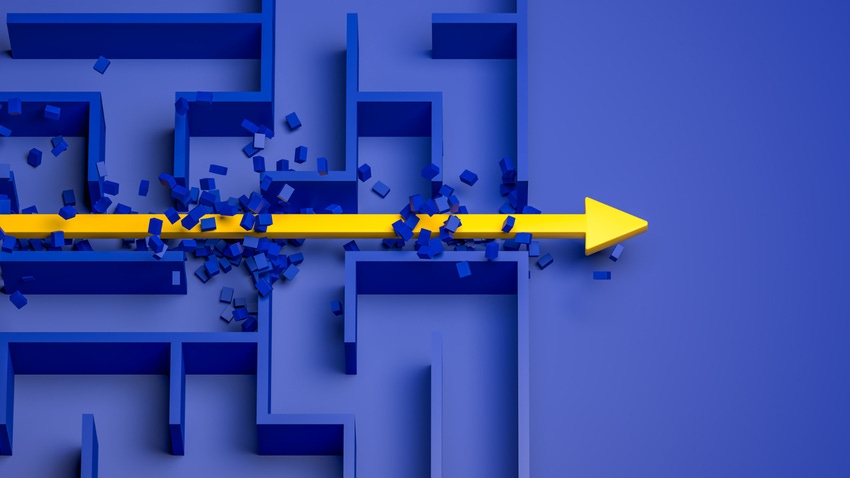
A recent Gartner survey of 2,500 executive leaders reports that 70% said their organizations are investigating and exploring AI deployments, while 19% are in pilot or production mode. On this basis, it is safe to say that the use of AI in business is booming as organizations increasingly seek to harness the power of this game-changing technology.
However, rather than diving straight in, launching a successful AI project requires careful consideration of the objectives, data requirements, and related ethical considerations. By understanding the potential pitfalls and adopting best practices, business leaders can turbocharge and operationalize their data and AI initiatives.
Define the purpose, use the right data
Before delving into any AI project, it is essential to define the problem you want it to solve and identify the specific purpose of the AI software program. AI should be viewed as a means to address a specific objective, rather than as a technology in itself.
Stakeholder involvement is crucial in ensuring that the AI program aligns with expectations and delivers actionable outcomes. Additionally, having skilled professionals in data science and AI is vital for evaluating the feasibility of AI solutions and determining the necessary data requirements.
AI project launch: Ethics and legal considerations
Obtaining the right dataset, whether collected purposefully, provided by third parties, or pre-existing, is key to achieving the desired outcomes. But organizations must consider the role and importance of ethics and legal expertise before acquiring the data. For one, they must ensure they have the legal rights to use the data they acquire.
Furthermore, it is imperative to assess whether AI is truly needed to solve the problem at hand. Sometimes simpler approaches may suffice, which can save valuable time and resources.
Avoiding AI failures: Clear objectives and quality data
The potential failures of AI projects often stem from a lack of well-defined objectives and insufficient or poor quality data. It is therefore important to involve data scientists, AI engineers, and outcome stakeholders from the project's inception. Through close collaboration, organizations can ensure the objective is well-defined, the right data is available, and the data quality meets the necessary standards. Taking a holistic view that considers technical, social, legal, and cost aspects is crucial for the successful integration of AI solutions.
Can you trust the AI?
Although AI usage is exploding in many settings, can businesses put their full trust in it to make important decisions? Organizations embarking on an AI project must ask themselves this question. The level of trust placed in AI should be considered in the context of the extent and potential impact of the decision’s consequences.
While certain decisions can be fully delegated to AI, others may require human involvement with AI assistance. Trustworthy AI requires human supervision during model training, continuous feedback from users to refine accuracy, and careful dataset curation to mitigate biases.
European regulations, such as the proposed AI Liability Directive, aim to ensure the responsible and trustworthy use of AI, further emphasizing the need for comprehensive AI project management and appropriate diligence.
Connect AI applications to business models
AI software programs offer a wide range of services including optimizing business models and organizations, as well as introducing new services to customers and users.
But it is important to assess their relevance and performance by monitoring and assessing the accuracy of their results. This ongoing evaluation helps identify if the services delivered meet the needs of users with the right quality or if they serve the organization as expected.
At the base level, this will validate the overall architecture implemented by the AI application. At a fine-grained level, this feedback loop may facilitate the collection of more data to continually train and enhance AI models. Entering this virtuous circle is key to gaining adoption of AI applications by users and will lower the cost for their exploitation and maintenance in the long run.
Business communication is a domain where AI services bring a lot of value by streamlining, securing, or enriching the real-time interactions among all stakeholders. The validation of the relevance and the quality of the service is straightforward and directly linked to its usage.
In addition, the operation of the service will, by essence, generate a continuous flow of data that can be easily collected and aimed at continuously improving services, making them more robust and efficient.
The evolving regulation of AI
Regulation in AI focuses on privacy, data sovereignty, and ensuring the ethical use of data. With data privacy and protection gaining significant attention, solutions must be designed to comply with national and specific regulations.
The EU's approach to trustworthy AI, privacy protection, and certification requirements impacts the industry, driving the need for adaptable network and data architectures. These evolving regulations, while presenting challenges, also open new opportunities for organizations to offer hybrid AI solutions tailored to customers' preferences.
Future-proofing AI initiatives
In the fast-moving fields of AI and data science, future-proofing initiatives requires building and retaining skilled tech teams capable of bridging the gap between technology and business objectives.
Developing a culture of AI adoption across the organization enables a holistic understanding of the risks and opportunities in discussions with customers. Additionally, knowledge-sharing with other industries through participation in events and other platforms fosters innovation and helps organizations stay ahead in the AI landscape.
Conclusion
The success of data and AI initiatives hinges on careful planning, stakeholder involvement, and the right expertise. Organizations must refine their objectives, leverage the appropriate data, and consider ethical and legal implications. By addressing potential pitfalls such as objective definition, data quality, biases, and risks, businesses can establish trustworthy AI practices.
Continuous improvement, benchmarking against success metrics, and integrating AI applications into business models drive maturity and alignment. Adapting to evolving regulations and future-proofing initiatives require building skilled teams, fostering knowledge-sharing, and developing tailored AI solutions. With these insights and best practices, organizations can navigate the AI landscape and unlock the true potential of data-driven decision-making.
About the Author(s)
You May Also Like