Context is King: Moving beyond BI to uncover the value in your data
With so much data being collected and stored, we’re now at a tipping point where we need more than data visualization to help make sense of it all
February 25, 2021
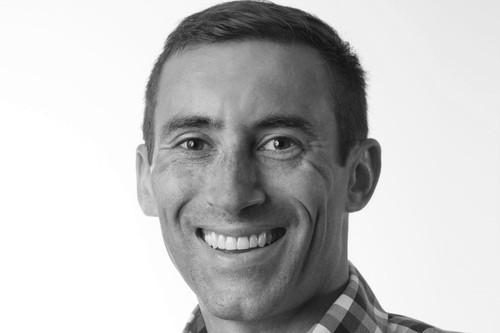
Sponsored Content
Organizations invest in big data and business intelligence (BI) tools to collect, store and visualize vast amounts of data to drive informed decision-making.
Compared to even a few years ago, the ability to see trends and changes in large, consolidated data sets has grown substantially.
But, despite steps forward, challenges in identifying the value within the data still exist.
And it comes down to this: the more we dive in to understand our data, the more we realize there’s so much more we could learn from it, if we only had the tools.
With so much data being collected and stored, we’re now at a tipping point where we need more than data visualization to help make sense of it all. This is not to say that BI visualizations aren’t beneficial. They are. With a BI platform, we can turn raw data into helpful information through dashboards, graphs and charts. We can drill down into data sets and see changes in data over time, by audience, by channel. We tell BI what we want to see visually, and it shows us. But that’s where it stops. BI tools haven’t yet met the demand to analyze what is happening in all that data to provide the insights needed to become agile.
Answer my question
What we’re learning through the adoption of BI is that BI’s greatest achievement is also its limitation. It only reveals part of an organization’s story. This is because BI can only regurgitate back to us what we ask of it.
For example, a data analyst at a retail brand might want to understand sales of certain SKUs by region over a designated amount of time. A visualization might show a downward trend in regional sales, which could then prompt big changes to the organization’s planned regional marketing campaigns. But what it won’t show a data analyst or marketing leader, is that this downward trend was due to changing buying behaviors in the region that have started to carry over into other regions.
Unless the analyst looks specifically at the regional drill down, then identifies the one city, store and changing purchasing behaviors, beneficial context can be easily overlooked or lost in an aggregated data visualization. For retailers with hundreds or thousands of stores, this kind of investigation doesn't happen. In fact, an unguided search approach for data-driven insights is not only time consuming, it’s nearly impossible given the volume of data.
The takeaway is that looking at sales trends using visualization alone is unlikely to identify the significance of an increase or decrease because there’s no context or expectation of what the standard model of behavior should be. So, unlocking true value from the data requires more than visualization.
To unlock it, we need to understand the expected model and the context around the data. This is what enables retail marketing teams to shift or optimize promotions – for example switching from business wear to yoga pants, or from in-store promos to online – having an immediate impact on potential conversions.
Tell me what I don’t know
BI was not designed to autonomously uncover unusual, unexpected or interesting activity in data and float questions back up to an analyst. But, that’s the next stage of enabling intelligence and it’s exactly what we need. When we don’t identify the right challenges, organizations are left with nice looking charts and graphs that don’t showcase the underlying business challenges or opportunities. So, when we apply intelligent, automated tools to the same data that BI is using for visualizations, we suddenly create a new way to view, understand and apply our data to solving real-world problems.
AI-driven automated business analysis (ABA) platforms – sometimes called augmented analytics – are designed to run in parallel to BI platforms and use the same data that BI uses. They can be set up in minutes to analyze data where it’s stored, eliminating extensive deployment times and the wait time typically needed for a platform to start monitoring for aberrant behavior.
If behavior changes from what was expected, the platform automatically surfaces the change to an analyst, marketer or other decision maker. Unlike anomaly detection, which similarly alerts based on changes from known or programmed parameters, ABA requires no pre-set thresholds, allowing it to alert on changes no one expected.
This means that organizations can identify and discover ideas in data where analysts never thought – or had the ability – to look. AI sifts through data continuously and finds unexpected behaviors quickly. This happens automatically and in real time by consolidating data and performing the deep analysis needed to help businesses make more informed decisions.
AI plus human intelligence creates new value
ABA doesn’t alleviate the need for analysts and data scientists. It simply elevates areas that require more attention, allowing analysts and data scientists to focus their attention on the most important issues and help the organization make decisions that can quickly impact business performance. In organizations with limited, or no available analysts and data scientists, ABA’s reported “stories” are simple enough for marketing, supply chain, merchandising and other line-of-business managers to understand and leverage in their daily planning.
When faced with huge amounts of data, BI tools help paint one part of the business picture. But organizations need to consider expanding their toolsets and embracing AI to see the full picture. ABA platforms should be considered an enhancement to BI, not a replacement, as each has its unique value. Designed to seamlessly integrate with myriad data sources, including cloud-based APIs and on-premise data sets, ABA can tap into a rich set of data and is ideal for fast-changing data. Organizations using BI tools, but still searching for the next level of business insights and value, are starting to apply intelligent AI to their data sets, creating more agile marketing-driven organizations and helping maintain a competitive edge.
As vice president of analytics at Outlier, Doug Mitarotonda focuses on the convergence of data science, analytics and economics. His academic and professional experience have led to a lifelong interest in the environment, climate change and data analysis. Learn more about Doug here.
You May Also Like