Context-Ready Algorithms Are Critical For Ethical AI
Context-Ready Algorithms Are Critical For Ethical AI
March 15, 2019
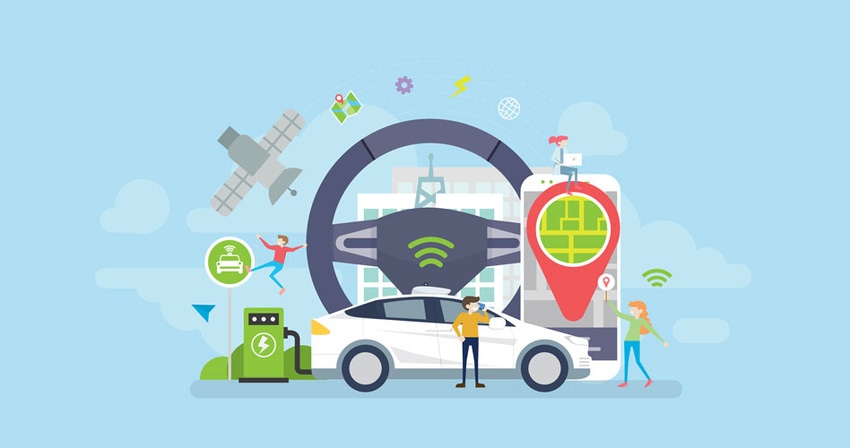
by James Bell
NEW YORK - Imagine the scenario: a driverless car is in trouble and is about to crash. It can plunge into the people in lane one, or it can swerve and hit the people in lane two. It has only seconds to decide which path to take.
To seek the answers to such challenges - known as trolley problems - has been the domain of philosophers and ethical scientists for generations. One bid to devise a way of teaching computers to answer this challenge was attempted by the MIT Moral Machine, where a crowdsourced approach asked the public to play an onscreen game to determine whom the car should hit - presumably to decipher how humans in the aggregate might answer this issue.
They answered it badly.
The problem of using aggregated human responses is that humans are walking examples of bias. We all have lives with a dual perspective, that of inside and outside, us and other.
This duality naturally leads to humans putting their own life first and working out from there to family, friends, neighbors and country in a layered approach.
These layers represent our attachments. From there all sorts of bias will arise that, from a distance, looks like prejudice against those in the outer layers versus those in the inner ones.
Related: Trust In Big Data Matters For AI - Here's Why
Bias in data makes biased AI a certainty
Training a mathematical algorithm from such a nest of complexity—an algorithm designed and superlative at finding correlations in any data set—is to not only discover and repeat that bias; but, in many cases to exacerbate it many times over. I once asked a data scientist if it was possible that bias in the data set will result in bias in the final model. She replied that, no, it was not possible; it was certain.
The standard response to this is to blame the data, with the reason for the poor performance by a machine learning model placed firmly at the feet of the training set. However, this misses another important point: context. Context is how a human determines elements related to those under consideration, how a human determines 'why'.
A machine learning classifier might be trained to determine that a person in a photo is throwing a frisbee. However, the context of why they are doing that, what a frisbee might feel like in the hand, the sound it makes through the air, etc. These are all questions the model cannot answer; but, any human can. Machine learning may be amazingly powerful, but it is also amazingly narrow in its 'intelligence'. For me, this earns it the moniker 'artificial' in a sense of the word not often applied to artificial intelligence.
Coding for context
How can we code for context? Consider our driverless car and its impending crash: What if the AI in the car used facial recognition to determine who the people in each lane were, then logged into their social media accounts and worked out who voted for whom? This nightmare scenario may not be so far-fetched as in China they are rolling out the 'social score' where a low score will impact your life directly, restricting such things as using planes for travel.
Consider then the reports of a well-known Chinese businesswoman receiving a jay-walking ticket via a facial recognition AI that monitors a crosswalk. When the lady contested the fine, a consultation with the tape showed that her face was on a poster affixed to the side of a bus as it went through the crosswalk at speed. The AI was unable to apply the context required to make the correct determination as its understanding was too narrow.
Related: Responsible AI Needs Radical Transparency
What can we do to prevent this? Currently, governments have reacted aggressively to protect private data from being used in algorithms or, at the very least, to insist on ethical design principles such as explainability and transparency.
However, this will not prevent examples such as the Louisville doctor who was dragged—bleeding—from an overbooked plane after refusing to leave when selected as the least valuable passenger (to the airline) by an algorithm.
The airline called this an "involuntary de-boarding situation,” a hollow terminology for what is putting the man’s patients at risk, all because the particular context of the situation was not a matter for the algorithm to consider.
For now, while the technological limitations of AI decision-making systems are causing such chaos, the argument is for humans to be brought back into the mix. Humans who can gainsay the algorithm and bring the human context required to defuse the situation successfully.
For me, this is the next logical step, one that we have seen before and perhaps it starts to answer the fears of those concerned that AIs are set to replace their jobs. For an example of this happening before, consider the advent of the computer. People feared computerization would cost jobs; but, they hadn’t accounted for the impact of the time it took to roll out the technology.
The cold-hearted mainframe computer became the “personal” computer; a friend, a companion with whom you could work to reach your common potential. Human and machine were augmenting each other. So, it could bear out for AI as well.
We spend a lot of time arguing about what AI means. Perhaps, like the computer, it will change to mean augmented, rather than artificial, intelligence - where the AI and the human can make good decisions together.
Only then will I feel more
confident crossing the road.
As Head of AI & Machine Learning at Dow Jones' Professional Information Business, James brings expertise from Financial Services, as an SME on Artificial Intelligence, Solution Design, SWIFT payments, Financial Compliance and Project Management. He has built, developed and led multiple teams in Anti Money Laundering and Data Quality, globally. As an AI thought leader, James has published webinars, articles, training courses and public speaking engagements.
About the Author(s)
You May Also Like