August 23, 2019
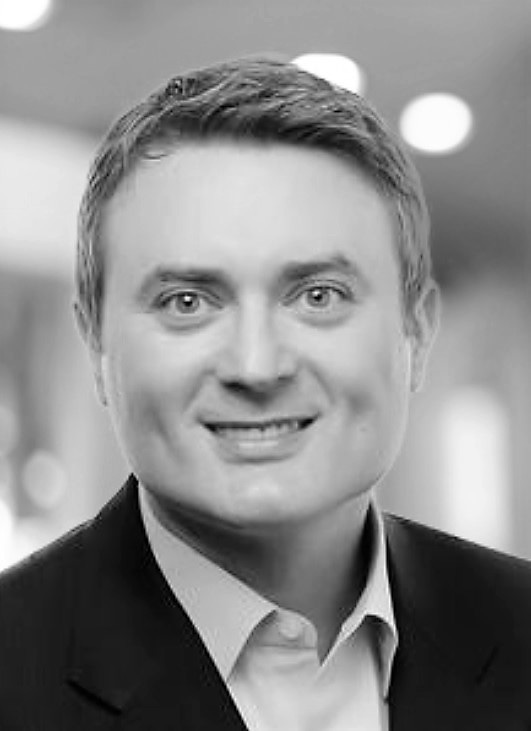
The cost of getting AI wrong extends beyond the financials—lost revenue, fines from compliance failures—to reputational, brand, and ethical concerns
by Martin Sokalski 20 August 2019
Key business decisions at scale have a
determining effect on success; as an example, should we approve a credit card
for a customer?
Among the decisions for each customer: the annual percentage rate, the spending limit, and a long list of other factors. Machine learning models are typically making these decisions for millions of customers.
In a very real sense, given the scale, the business is in the hands of a handful of smart data scientists—and the machines they build and train—using ground truth created from historical loan data.
Autonomous
algorithms: then vs. now
Most algorithms today are relatively simple
and deterministic: they produce the same output from a predetermined set of
states and a fixed number of rules. The approaches for evaluating them for
validity and integrity are largely established and adopted. In fact, in our
estimation, over 80 percent of the leading practices needed to maintain their
accuracy and effectiveness are known.
Think of expert systems in manufacturing.
Think of actuarial science that uses deterministic rules or decision tables in
insurance. Think of robotic process automation in financial services.
It isn’t that hard to determine whether the
conclusions they reach are acceptable—and sound and scalable supervision is
relatively easy.
These rules can get very complex,
especially when the number of attributes (also known as features, or variables)
in the data or the number of records increases.
Machine learning and deep learning—and
other types of AI—are creatures of a different kind. They are trained to learn
from data (commonly referred to as ground truth) instead of being explicitly
programmed, which means they can “understand-learn-uncover” the nuances and the
patterns in the data, they can handle a very large set of attributes, and are
often significantly more complex in how they do what they do.
Think of training a prediction model from a
set of a million past loan applications, which in turn uses 100 attributes.
Think of detecting a tumor from a million MRI images. Think of classifying
emails. Once trained and evaluated, these models can be provided with new or
unseen data from which they can make predictions. They are probabilistic in
nature and respond with a degree of confidence.
While all of these aspects are good, it can
be unclear what the models are doing: what they learn, particularly when
employing opaque deep learning techniques such as neural nets, how they will
behave, or whether they will develop unfair bias over time as they continue to
evolve. That’s why understanding which attributes in the training data
influence the model’s predictions has become very important.
Algorithmic
risk: trust in the machine
Let’s take a closer look at a potential
problem for the business leader in the loan division of a big financial firm.
If an error hides within an algorithm (or
the data feeding or training the algorithm), it can influence the integrity and
fairness of the decision made by the machine. This could include adversarial
data or data masking as ground truth.
The business leaders are on the hook for
preserving the brand reputation for the firm, even as the AI models
increasingly make decisions that might not be understood or in line with
corporate policies, corporate values, guidelines, and the public’s
expectations. Multiply these issues by the number of algorithms the loan
division is utilizing. This is when trust weakens or actually evaporates.
Keeping AI in check
A number of techniques, including those
based on renormalization group theory, have been proposed. As models
across AI tasks—including computer vision, speech recognition, and natural
language processing— become more sophisticated and autonomous, they take on a
higher level of risk and responsibility. When left untrained for long periods,
things can go awry: runtime bias creep, concept drift, and issues such as
adversarial attacks can compromise what these models learn. Imagine compromised
MRI scans or traffic lights being manipulated in a smart city.
Continuous-learning algorithms also
introduce a new set of cybersecurity considerations. Early adopters are still
grappling with the magnitude of risks presented by these issues on the
business.
Among the risks are adversarial attacks
that hit the very foundation of these algorithms by poisoning the models or
tampering with training data sets, potentially compromising privacy, the user
experience, intellectual property, and any number of other key business
aspects. Consider the impact on lives or an environment of an adversarial
attack in medical devices or industrial control systems. Tampering with data
could disrupt consumer experiences by providing inappropriate suggestions in
retail or financial services. Such attacks might ultimately erode the
competitive advantage that the algorithms were intended to create.
With complex, continuous-learning algorithms, humans need to know more than just the data or attributes and their respective weights to fully realize the implications of the AI getting it wrong or going rogue; they need to understand aspects such as the context and intended purpose under which the model was developed, who trained them, provenance of the data and any changes made to it, and how the models were (and are) served and protected. And they need to understand what questions to ask and what key indicators to look for around an algorithm’s integrity, explainability, fairness, and resilience.
This
opinion was originally published as part of Controlling
AI, a KPMG research campaign investigating responsible design and
operation of AI programs.
Martin
Sokalski is a global leader for KPMG’s Emerging Technology Risk practice. He
helps organizations around the globe embrace the “art of the possible,” enabled
by emerging technologies like artificial intelligence, by facilitating
ideation, innovation, and responsible adoption.
Martin
regularly speaks at conferences and contributes to thought leadership on
artificial intelligence, digital transformation, and emerging technologies. He
believes that adoption of AI at scale is currently inhibited by lack of trust
and transparency, explainability, and unintended bias and aims to work with
industry leaders to solve for that challenge.
About the Author(s)
You May Also Like