Just as Netflix continues to power its entire business on the data it gathers from its enormous subscriber base, retailers should be looking to leverage the information readily available to them.
July 26, 2021
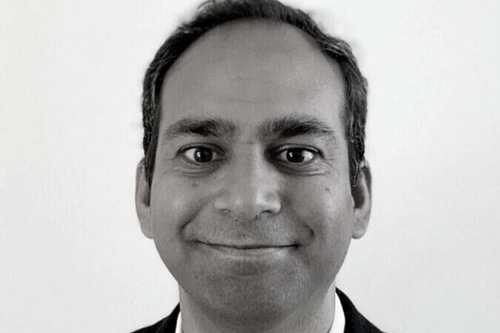
Sponsored Content
Netflix is, without doubt, one of the most adept corporations in the world when it comes to extracting value out of data.
Its entire business model is underpinned by the information it gathers from its subscriber base, which in Q1 2021 comprised of well over 200 million people.
From viewing habits and preferences to search history and basic demographics, the streaming platform has mastered the art of personalized experiences.
This enables it to retain customer loyalty – crucial for the longevity and financial sustainability of the business.
The COVID-19 pandemic provided Netflix with something of a subscribership boom, and although the growth levels of 2020 appear to be slowing, Q1 still saw the organization take in record quarterly revenues of $7.16 billion.
Retailers too can boost their bottom lines by making the most out of the data available to them.
By collecting and analyzing data effectively, retail enterprises can not only enhance their in-store and online offerings but also make smarter, more informed decisions that can lead to a greatly enhanced customer experience.
Indeed, it can easily be argued that data holds the key to retailers retaining customer loyalty – something which has become notoriously difficult to do in the age of multichannel purchasing.
Unobtrusive data collection to fuel AI
What do customers buy? When and where do they buy products? What offers are taken up the most? These are just a handful of the fundamental questions that savvy data collection and analysis activities can help to answer, and Netflix has mastered the art.
Most of Netflix’s customers will not recognize the algorithms and data collection techniques it uses. As they browse and watch content, the platform learns by tracking what is searched and watched, when specific shows are watched and how highly they are reviewed.
Retailers can also adopt unobtrusive data collection strategies.
As a starting point, they can use payment data to gain a breakdown of what a customer buys in-store (and online) – information which could inform what products to prioritize for valuable shop floor space.
Sensors, cameras, and smartphones are also data sources that physical retail stores can extract valuable information from.
From recording the path customers take around the shop floor and scanning what they browse to the frequency and timing of visits, there is a lot of data that can be harvested by the clever use of artificial intelligence and Computer Vision (CV) technology.
The field of CV most relevant to retail is temporal activity recognition (TAR). This enables segmentation and detection of objects in 3D space, meaning retailers can identify items being picked up and by who – importantly, it can detect if products are being stolen.
Amazon has already taken Computer Vision and TAR to another level with the launch of its checkout-free stores, the first outside of the US recently being opened in London.
Another use case of CV centers around stock management and product identification. Here, Computer Vision could be used to help retail staff respond to queries about whether a certain product is in stock. Meanwhile, the ability to monitor shelf stock levels and apply live in-store analytics on the edge will allow stores to replenish key products at the earliest opportunity.
Going further, CV on Internet-of-Things (IoT) Edge devices can integrate real-time stock data with customer demand analytics models to trigger orders with suppliers.
Furthermore, it also empowers retailers to stock products that their customers actually want to buy.
The simplest of retail concepts, it is more important than ever to nail product offerings given the enormity of choice consumers are faced with both in-store and online.
This lies at the heart of Netflix’s success to date. Because of its formidable collective understanding of its subscriber base, the platform can strategize its content calendars with a high degree of confidence.
Its track record of releasing highly acclaimed shows is no coincidence – The Queen’s Gambit, for example, was one of the most-watched series in 2020.
Using AI to personalize the customer experience
Loyalty and reward cards are other highly effective data gathering tools that can enhance the end-user experience.
In the online retail sphere, consumers are bombarded with product advertising and messaging across numerous channels – via apps, emails, social media, website pop-ups, and more.
Creating a truly personalized and engaging experience is therefore critical, and this is where AI and deep learning can power loyalty programs to maximum effectiveness.
Deep learning enables the recording and analysis of customer behavior such as product views, store visits, purchases, ratings, reviews, and referrals.
Using this information in combination with metrics such as gender, age, location, and marital status, retailers can create segments that allow them to offer more personalized loyalty and reward experiences. UK supermarket Sainsbury’s, for example, offers unique Nectar point bonus rewards on items carefully selected for each customer based on their purchase history.
One of the clearest ways to see how Netflix leverages segmentation is through its highly personalized dashboard or home screen that is unique to every account holder and user.
It presents content in individualized lists such as ‘Watch It Again’, ‘My List’, and ‘Top Picks’, as well as genre catalogs based on what type of movie or program users typically opt for.
Back in the retail arena, Amazon is arguably the greatest exponent of personalization. More than a third of its revenue is generated via its recommendation tool, an engine powered by user data that can deliver huge upticks in earnings even with minor algorithmic improvements.
In the brick and mortar world, if you walk to a Dior store anywhere in the world, the shop assistant will know which exact shade of foundation you’re using.
Matching customers through collaborative filtering
Online fashion retailer ASOS is another master of personalized ecommerce.
One technique it uses to great effect is collaborative filtering. The end result allows ASOS to find customers with similar purchasing habits and recommend products that other like-minded shoppers have already bought.
However, the process is more complex than segmentation. Collaborative filtering is a form of matrix factorization, and even by ASOS’s own admission, has its blind spots.
When a new product is introduced, it takes some time for ASOS to obtain a large enough number of customer interactions to recommend it – given that 5,000 new products are introduced every week, this presents a problem.
To navigate this, ASOS adopts what it calls a hybrid approach which leverages customer-product interactions together with the augmented product attributes to provide recommendations to customers.
This, along with other personalization features such as automated sizing recommendations, has helped to propel ASOS into one of the most trusted online-only retailers.
And as the world of retail continues to evolve into a multichannel environment where customers interact with brands and businesses both physically and (increasingly) online, those retailers that can capture, analyze and leverage the power of data will no doubt fare better than those which do not.
Just as Netflix continues to power its entire business on the data it gathers from its enormous subscriber base, retailers should be looking to leverage the information readily available to them.
Technologies and techniques such as Computer Vision, deep learning, and collaborative filtering represent some of the ways retailers can transform into data-driven enterprises.
Jai Gandhi is VP of data and analytics at Ciklum. He focuses his work on finance, procurement, and data functions, particularly listed companies in the retail, consumer packaged goods, and media industries.
You May Also Like