Adopting a holistic approach and proactive data management strategy are key to ensuring data quality.
December 8, 2022
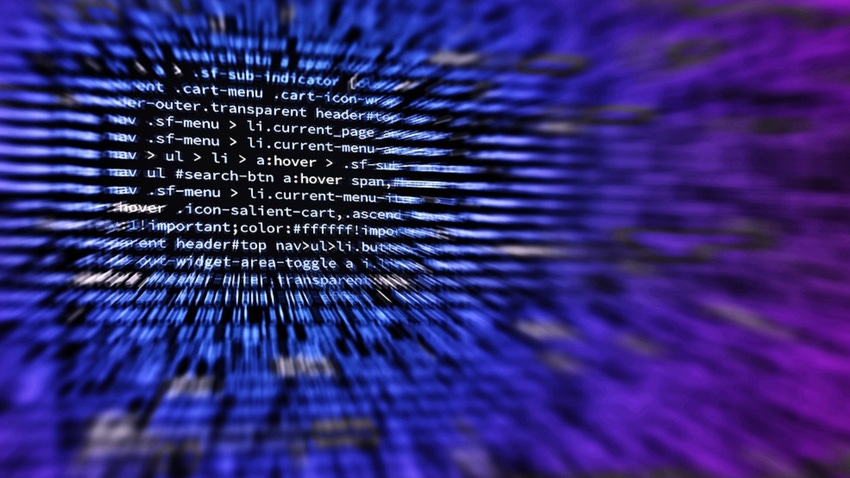
Sponsored by IBM
Throughout 2022, a clear theme has emerged among business leaders we meet. They are eager to digitize and have their business and IT teams embrace AI and automation, and they want it to happen fast.
But there are several emerging challenges that are getting in the way of this goal, including an evolving regulatory and compliance landscape and macroeconomic issues like inflation, demographic shifts, talent shortages, supply chain bottlenecks, and so much more.
Technology has the potential to help businesses navigate these obstacles, and when I get asked for advice on where these initiatives should start, my advice is always that you must begin with quality data.
AI and analytics can help drive informed business decisions, and it’s no secret that good business decisions start with a foundation of data that you can trust. When it comes to data, trust means delivering a comprehensive view of quality data that is governed and ready for analysis. But we often hear that data quality is a barrier that businesses are facing in their journey to become more data driven. The consequences of data quality issues can be far reaching, here are three examples:
First, no amount of AI or algorithmic sophistication can overcome poor quality data. AI projects could be quickly sidelined or derailed if you don’t have a curated set of high-quality data to begin with.
Data governance is becoming even more critical within enterprises, and effective governance initiatives require data quality. With data increasingly sprawled across multiple different environments, it’s easy for data to become siloed and for poor quality and inconsistent data to flow downstream to your data users, jeopardizing the integrity of applications and models.
Finally, data quality issues can result in unnecessary firefighting from already stretched data engineering teams. Data quality issues tend to compound over time. They can be difficult to spot and can easily go unseen for days, which can result in costly business errors or damage to brand reputation. If poor quality data has already moved down stream, it’s even harder for data engineers to fix the issues quickly.
Maintaining data quality is easier said than done so a clear strategy that relies on proactive data quality management as data moves from producers to consumers is key. It’s also important to embrace approaches that enable data quality in the first mile as early as ingestion, including active monitoring and automated data cleansing at the source.
Additionally, innovation in metadata and intelligent automation can also driving improvements in data quality. The use of active metadata can help foster greater understanding and trust in data, as well as help organizations get the right data in the right hands at the right time. Equally important is the ability to understand data lineage by being able to track the flow of data back to its original source, which is important for governance initiatives and helps data engineers when they are fixing data quality issues.
As businesses look ahead to 2023, we believe AI and automation initiatives will continue to serve as a potential competitive advantage. Prioritizing a strong data strategy and architecture, including data quality, can help drive these initiatives towards success.
Learn more about IBM’s holistic approach to data quality here.
Read more about:
Partner PerspectivesAbout the Author(s)
You May Also Like