Deploying Multimodal AI? Test Its Reliability with MMCBench
New MMCBench test will let businesses evaluate multimodal model reliability before deployment, even with noisy real-world data.
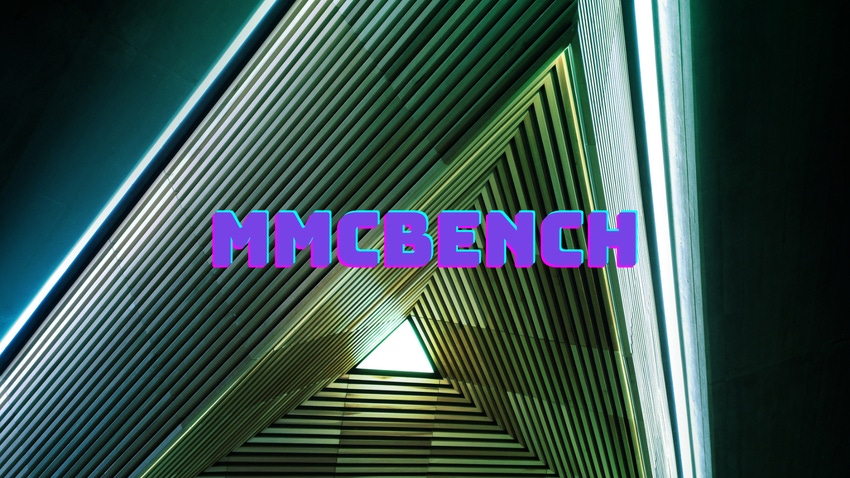
At a Glance
- Businesses can now evaluate the real-world robustness of commercial multimodal AI systems with new benchmark.
A new benchmark test will let businesses inspect the reliability of commercial multimodal AI models when they are fed imperfect, noisy data.
MMCBench was created by researchers from Sea AI Lab, the University of Illinois Urbana-Champaign, TikTok parent ByteDance and the University of Chicago. It introduces errors and noise into text, image and speech inputs, then measures how consistently over 100 popular models like Stable Diffusion can generate outputs.
The new benchmark spans text-to-image, image-to-text, and speech-to-text, among others. It would allow users to determine if multimodal models are more trustworthy and robust when data gets corrupted – which could help businesses avoid costly AI failures or inconsistencies when real-world data does not match training data.
MMCBench involves a two-step process – first, selection, which determines the similarity. Non-text inputs like model-generated captions or transcriptions are compared with text inputs before and after corruption. Then, the evaluation process measures self-consistency by comparing clean inputs with outputs from corrupted inputs.
The resulting process provides users with an effective tool to evaluate multimodal models. An overview of the MMCBench process can be seen below.
Multimodal models are becoming increasingly prevalent in the AI space, however, there are limited tools for developers to evaluate these emerging systems.
“A thorough evaluation under common corruptions is critical for practical deployment and facilitates a better understanding of the reliability of cutting-edge large multimodal models,” a paper outlining the benchmark reads.
The MMCBench project aims to address the gap. It is open source and can be used to test commercial models. The benchmark can be accessed via GitHub, with the corrupted data available on Hugging Face.
The benchmark does have some limitations, however. For example, the use of greedy decoding during evaluation, where the token (word) with the highest probability as the next token in the output sequence is chosen, could underestimate the true capabilities of some models. Also, high output similarity could mask poor-quality results.
The team behind the benchmark, however, plans to update new models and add more modalities, like video, into MMCBench, so it should improve over time.
Read more about:
ChatGPT / Generative AIAbout the Author(s)
You May Also Like