Quantum-Powered Machine Learning Spots Pneumonia in Chest X-Rays
New method works faster and makes fewer mistakes than comparable techniques
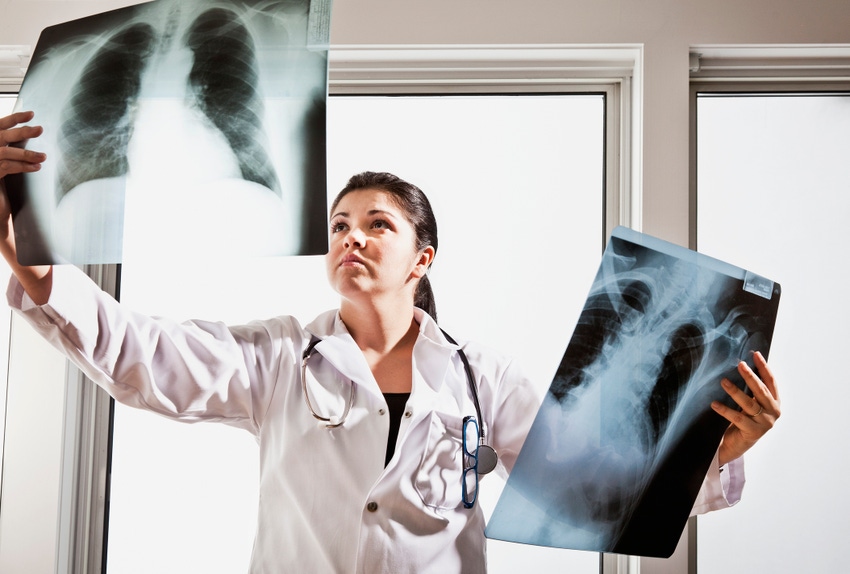
Researchers have combined quantum-inspired computing methods with machine learning to better diagnose pneumonia from chest X-ray images.
Pneumonia is an infection in the lungs that can cause a cough, difficulty breathing, a high temperature and chest pain, with severe cases needing hospital treatment.
It is most commonly diagnosed by radiologists who examine X-rays looking for white spots on the lungs called infiltrates that indicate an infection.
However, long backlogs and workforce shortages mean that getting a diagnosis early enough for treatment to have the greatest chance of success can be challenging. According to the World Health Organization, pneumonia accounts for 14% of deaths in children younger than five years old.
A team led by Carnegie Mellon University professor Sridhar Tayur has published a paper detailing a study that shows that applying quantum computing techniques to machine learning can detect pneumonia in X-rays quickly and accurately.
The method uses an approach called binary classification, teaching the system with images that show signs of a pneumonia infection and those that don’t so it learns to distinguish between them.
The researchers used a class of algorithm called support vector machine for classification using quantum-inspired computing. Compared with other classical, quantum and hybrid approaches, it made fewer mistakes and took less time to make a diagnosis.
Tayur founded the Quantum Technologies Group at Carnegie Mellon to better understand and apply quantum computing methods to industries such as health care.
The researchers proposed that the method could also be used to diagnose cancer.
This article was first published in AI Business's sister publication Enter Quantum.
About the Author(s)
You May Also Like