The Road To Artificial General Intelligence: In Conversation With Ben Goertzel
October 23, 2018
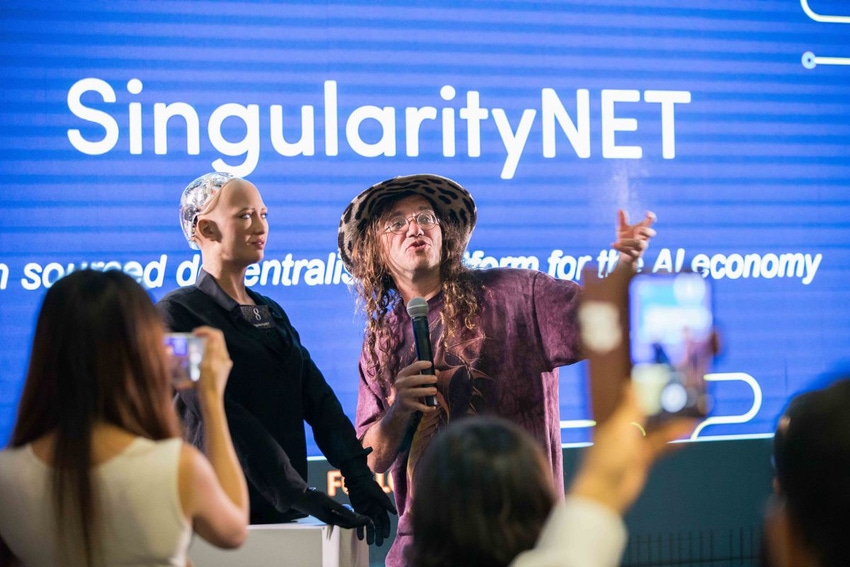
By Ciarán Daly
HONG KONG - Futurist, Hanson Robotics Chief Scientist, and SingularityNET CEO Ben Goertzel is a busy man, to say the least. Off the back of a bumper year for Hanson Robotics' AI figurehead, Sophia, Goertzel is currently right in the middle of preparation for the beta launch of SingularityNET, a blockchain-powered decentralised marketplace which has the dual goals of delivering AI tools to customers while slowly building artificial general intelligence using what he calls an 'eccentric' back-end.
Meanwhile, Goertzel is also working on a number of basic AI tools and services to include with SingularityNET, and tied to this are some new language and vision functions for the SOPHiA robot which will leverage SingularityNET features upon launch. It’s 11PM for him when we speak, and it doesn’t seem like his workday will be ending any time soon after our interview.
The following transcript has been edited for brevity and clarity
Q: Tell me about SingularityNET. It’s an interesting case study for the synergies between AI and blockchain—what are the key use cases?
B: “There’s two aspects to it. Firstly, we’re building a platform which anybody can use to create AI services and sell them to others. This platform is, by nature, decentralised—blockchain is used to enable decentralised control encryption and identity management and so forth. Instead of programmers just putting their code on Github, we’re hoping this can grow into the de facto way that people create and distribute new AI algorithms and services. On top of this, we’re putting some basic AI algorithms on there that everyone can use, and then we’re also building some more focused vertical market services on top of these.”
“Secondly, the main motivation behind SingularityNET is that this is the infrastructure I’ve wanted for my own commercial and R&D work in AI. I wanted a way to easily connect together multiple different AIs with one another in a flexible way, so that they could interoperate and perform a sort of meta-AI. I have this architecture for developing artificial general intelligence, OpenCog, which is a lot of deep algorithms working together to deliver abstract transfer learning and generalisation, to which you can add other people’s tools that include machine vision and other narrow functionalities. This often happens – you realise that a tool which makes sense for you to use in your own work could be opened up to a lot of people with a need for this sort of platform.”
Q: What are some of your priorities for SingularityNET in the next year?
“One of the realizations we came to is that it’s not enough to launch a great platform with a bunch of AI algorithms on it. We need to take it to the next level by creating vertical market-specific AI services, as well as the back-end to these algorithms and this platform. So we’re going to launch some quite specific services, starting with fintech, biopharma, mobile, and the automotive industries and so forth.”
“From there, it becomes a matter of soliciting customers for these services. To see adoption, the fact you have really amazing, novel algorithms and structures and protocols in place in the backend and the fact you’re decentralized isn’t good enough—you have to take it all the way to the customer. The product needs to be great, really easy to use, and solve customers’ specific problems. On the back-end, you’re using specialised products to fuel the emergence of more general intelligence on the back-end."
"That’s the architecture: we’re putting together the basic platform and protocol for a network of AI algorithms that is self-organising into a federation of algorithms with its own emergent intelligence, and then offering nitty-gritty, high-quality AI-based products aimed at specific classes of customers. Once we have large scale, we can benefit from the network effects between producers, consumers, and AIs—but to get there initially is black magic, as with every platform, and that’s gonna be our challenge in the next couple of years.”
Q: In the past, you’ve talked about SOPHIA as a ‘good platform for artificial general intelligence R&D’. Do you see SingularityNET in the same way?
B: “The SingularityNET platform can be used for narrow AI applications, or AI with a high level of general intelligence. Someone could offer an AI that does nothing but spot errors in accounting records and flag potential anomalies. That’s a super narrow use of AI, but it could make money for someone on the platform.”
“On the other hand, I believe SingularityNET could also be an excellent platform for creating general intelligence using hybrid architecture. This could use one algorithm for vision, one for hearing, one for mathematical reasoning, one for episodic memory, and connect all of these together. Many of the same tools that can be used for narrow applications can be used for lower-level AGI R&D—as well as long-tail applications that aren’t really covered by big tech companies.”
“That’s one of the beauties of this decentralized platform—you can address the long tail better than if things are operated by a centralized company with a fairly limited and specific set of applications that are consistent with their business model.”
Q: On that point, is there a problem with centralization of AI solutions across the sector as a whole?
“There seems to be. Whether there’s an intrinsic problem with it is a… philosophical question. But in practice, absolutely! There’s a huge amount of work going into training, say, face recognition models, and very little going into training models that will recognise disease in a farmer’s plants. Which of those is actually more useful, though?”
“On the algorithm side, there’s an insane amount of effort going into optimising deep neural network execution and learning but that’s one among a huge list of different AI approaches and algorithms that are in the AI literature—the industry has clung onto a narrow class of algorithms.”
“Another factor is that some AI methods are dependent on huge amounts of data and others, less so. Big tech companies that have a lot of data, however, have a strong incentive to focus attention on those algorithms which require a lot of data because that’s where they have a differential advantage. Research on unsupervised learning, one-shot learning, and general intelligence is sure to drift in the literature—not because they’re unpromising, but because a big company with a lot of money and a lot of data has less differential advantage for these types of algorithms.”
“The whole field then gets driven in the direction of what big tech companies are doing algorithm-wise, which is driven by where they have a differential advantage—rather than by what will solve the world’s problems.”
Q: So what application would solve the world’s problems? Where does AGI come in?
B: “Solving the world’s problems is a big task, right? You’re not going to do that with just one AI algorithm. You need to allow people to use their own initiative to solve the world’s problems. You want an ecosystem that encourages a young developer to figure out how to solve a problem—like diagnosing crop disease—and then make that AI become available to people around the world who want to use it. Our platform makes that easier.”
“To reach general intelligence, you need AI agents capable of highly abstract representation and abstract reasoning and learning, and you need to connect these with other AI agents which are doing more concrete data processing and taking specific actions for specific customers. Once you have the abstraction and generalisation areas of AI, you can connect them to the more concrete percept, action, and analytics areas. This network of AIs can self-organize into a sort of meta-AI and then you can get an emergent general intelligence.”
“However, it’s not like you could just put together a bunch of narrow, highly specialised AIs and merge them together and you’d magically, spontaneously have a general intelligence. You need some AI components which are specifically good at generalization and others with more specialized components. A sort of integrated general intelligence will be able to emerge out of that.”
“Of course, in the end, you can solve all the world’s problems by creating a benevolent superhuman AI that just builds a lot of stuff for you and stops bad things from happening—a benevolent robot ruler that sort of sits in the background and makes sure everything runs smoothly. That’s an end goal that interests me, but on the way there, it’s as much about creating a framework where humans can grow and connect their AI inventions together and they’re incentivised to do so, along with the programming of algorithms specifically oriented towards general intelligence.”
Q: You talk about this end goal of artificial general intelligence, but today, there’s already a lot of press around bias and issues in the datasets which are leading to unintended negative consequences. Won’t an AGI exaggerate those consequences further?
B: “Some of this is just a result of AI algorithms that aren’t as smart as they should be, right? You’re training an algorithm to recognise patterns from a certain dataset and it’s going to recognise what’s in that dataset, because AI algorithms aren’t yet able to understand abstract principles that may be ethical in nature or otherwise. It’s a limitation of AI: the specifics of the data. More general intelligence can certainly help there, and we need to focus on improving the smaller algorithms while we wait for the AGI to appear.”
Based in London, Ciarán Daly is the Editor-in-Chief of AIBusiness.com, covering the critical issues, debates, and real-world use cases surrounding artificial intelligence - for executives, technologists, and enthusiasts alike. Reach him via email here.
About the Author(s)
You May Also Like