Quantum AI Model Improves Early Cyber Threat Detection
New methodology uses adversary-generated threat intelligence to spot unknown cyberattacks
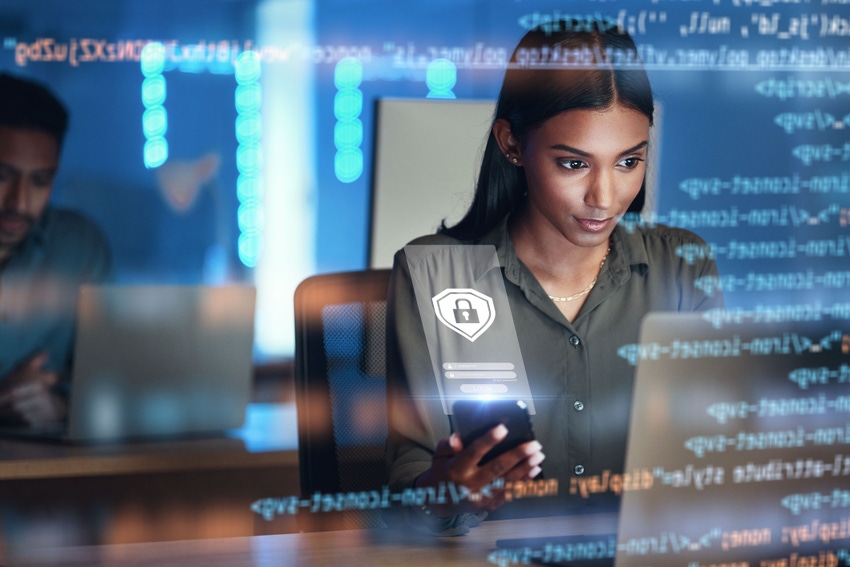
Researchers in quantum computing and cybersecurity have developed a machine learning model that improves the early detection of cybersecurity threats and increases the explainability of results.
The team, from quantum software company Multiverse Computing and threat intelligence specialist CounterCraft, trained a quantum AI model using datasets from actual network traffic and system logs that identified 100% of cyberattacks in a trial.
They used a methodology known as Matrix Product State (MPS). It uses adversary-generated threat intelligence captured during attempted attacks by hackers instead of traditional rule-based systems to identify cyberattacks.
CounterCraft analysts had already identified the attacks used in the training data, which they used as a benchmark to evaluate the performance of Multiverse’s new model.
During trials, the researchers found that the model reduced false positives more accurately than classical models. It also delivered improved the explainability of the algorithm’s results, a feature required by business users and regulators.
“Explainable AI supports robust decision-making by providing clear explanations for outcomes while improving understanding of threats and ensuring compliance with increasingly stringent transparency regulations,” said Multiverse Computing’s chief scientific officer Roman Orus.
“Our work with CounterCraft shows how quantum techniques can strengthen cybersecurity defenses against today’s threats and future ones while improving explainability.”
According to CounterCraft, a cyberattack generally consists of a series of between 20 and 80 individual events as hackers attempt to penetrate the system. The MPS model identified 83.5% of these steps as well as finding several steps missed in the classical analysis.
The model used real incident reports for training data covering various attack types, such as weak credential usage and exploits of known vulnerabilities. This enabled it to identify the abnormal behavior that signals the early stages of an attempted cyberattack.
“We provide total visibility into an attackers' tactics and techniques to help customers anticipate and understand the strategies used by cyber adversaries, and this new model based on tensor networks will improve those capabilities,” said CounterCraft CTO and co-founder David Barroso.
“The ability to detect unknown attacks both inside and outside the network is vital for early detection and response and is one of CounterCraft's strengths.”
The model also creates synthetic data that can be used for future training models and to simulate activity for deception strategies.
The team now plans to carry out further testing to enhance the model’s effectiveness in different scenarios.
The model could have future applications in other industries that depend on anomaly detection, such as finance, healthcare, government, critical infrastructure, manufacturing and retail.
This article first appeared on AI Business's sister site, Enter Quantum.
About the Author(s)
You May Also Like