2020 trends in data governance: the blueprint for controlling automation
2020 trends in data governance: the blueprint for controlling automation
October 14, 2019
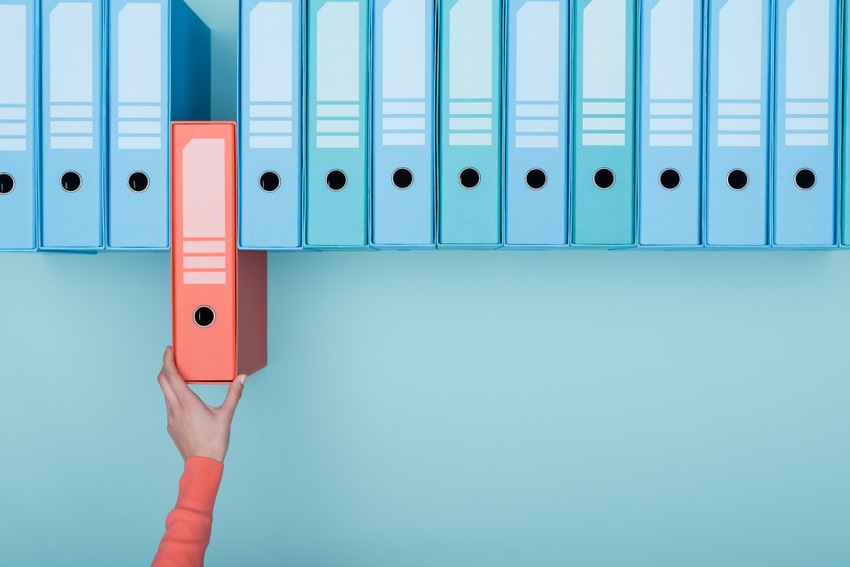
by Jelani Harper 14 October 2019
The full scope of the role of automation in the enterprise—it’s merits and drawbacks, rewards and risks—is most clearly evidenced in data governance. Processing decentralized data at scale would seemingly require some automation.
But conforming automation to the people, principles, and processes of prudent data governance can be exhaustive. Failure to implement any of these aspects could mean severe regulatory repercussions. The amount of data for training machine learning models, the autonomy of virtual agents, and expedience of Artificial Intelligence-infused actions only compounds the difficulty.
The crux of the situation is that for many facets of governance, “people are always looking to automate some of the processing,” Irene Polikoff, CEO of TopQuadrant, said. Oftentimes, those processes are required to simply understand data, its meaning to business objectives, and the optimal means of managing it from initial ingestion to disposal.
Consequently, organizations must learn to distinguish automation from automated decision-making. “Anything that involves making conclusions and making sure that things are correct, people need to be involved because the whole thing about governance and usage for compliance is a system of checks,” Polikoff noted.
Therefore, organizations must apply data governance staples of metadata management, provenance, lifecycle management, and data cataloging to contemporary developments in regulatory compliance, data privacy and more to automate data governance.
Automated agents and gamification
Many of the foundational elements of data management that require adequate governance can be automated with digital agents. These include:
Connecting data to sources: Automated agents can build connections between data and resources necessary for proper governance. Polikoff mentioned a use case in which data includes email addresses and agents can “build a connection to the business term that represents email addresses.”
Implementing data quality: Machine learning, Natural Language Processing, and automated agents are essential to de-duplicating data and implementing other facets of data quality at scale.
Inferring business rules: Automated agents can also “go after datasets and figure out some business rules based on the data,” Polikoff said. In real estate, for instance, digital agents can accelerate processing by analyzing loan applications to infer concrete rules for approval that are applicable to future applications.
There’s interest in leveraging gamification to implement the notion of human-in-the-loop vital to supervising such automation. Polikoff noted that gamification is useful as a "reward system or something that sort of builds a connection with bots or avatars.” Gamification is applicable to this and other aspects of data governance including data stewardship and lifecycle management because it engages data users. If users “get some rewards, you get some recognition, you get more of a community involvement so it’s more interactive,” she explained.
Regulatory compliance and data privacy
Although issues of regulatory compliance have expanded with specific mandates for data privacy, it’s critical to realize they simply accumulate atop traditional issues in heavily regulated industries such as finance or healthcare. Data privacy has become so eminent because it’s a horizontal concern with international developments, statewide initiatives, and proposed legislature at the federal level. Amidst the deluge of rules, it’s imperative that organizations conduct a comprehensive impact analysis to effect “governance of the entire set of regulations that might apply to you as a business,” said Robert Coyne, CMO at TopQuadrant. Relationship-sensitive approaches involving graph technologies are credible settings for “some kind of semi-automated way of sometimes doing at least rules-based inferencing to say, does this regulation apply in this location to this kind of transaction, or what are all the regulations that apply,” Coyne added.
Data classification
There are a number of ways to accurately classify data to stay on the right side of data privacy regulations. It’s often advisable to begin with a business glossary of relevant terms such as Personally Identifiable Information (PII), street addresses, emails, and other information. When it’s time to map the actual data elements to those terms, organizations can automate a variety of procedures pertaining to:
Data profiling: Although it may be possible to classify data based on document type or the repository they’re stored in, data profiling—running statistical analysis of data—eliminates most ambiguities about content and applicable governance policies. Automation of data profiling reveals the values in datasets. Once organizations connect to a database, “you get all the metadata: tables, columns, etc., and run queries over certain tables for statistics so you know how many rows are in a table, unique values in a column, whether it’s strings or integers or whatever,” Polikoff said.
Metadata management: Understanding metadata is implicit to data profiling; this understanding often provides the initial step in classifying data. Metadata is also helpful for tagging and cataloging data; machine learning can automate these processes. According to Rohit Mahajan, CTO of Io-Tahoe, cataloging data involves “the technical metadata, business metadata, and operational metadata. So if you have a column, let’s say an attribute called line_1, we would be able to, based on smart [data] discovery, call that out and tag it as a phone number.” Machine learning can do similar tagging for social security numbers, street addresses, and other PII.
Data sampling: On most occasions, organizations must do more than analyze metadata for accurate classifications, tags, and data cataloging. “The data and only the data will give you the most accurate and truest story,” Mahajan said. “All of our algorithms actually factor in the data itself and then do the prediction of the meta based on the data, as opposed to just [on] the meta.” Data sampling is a way of inputting small quantities into data cataloging tools to understand data sources in relation to governance mandates.
Provenance in motion
Metadata management is indispensable to establishing data lineage. It offers a roadmap of its journey through the enterprise—including transformations—as well as who may have accessed the data, and how. In this respect, metadata has always been essential for timely data integrations. However, as metadata itself becomes more prized by the enterprise as a means of generating business value, so does data lineage. Traceability is not only a means of taming the boundlessness for which self-service can potentially eschew governance requirements, it also benefits automation—whether in the form of machine learning, virtual agents, or recommendations.
Governance frameworks must “give me the ability to go back to something before,” explained Piet Loubser, SVP of global marketing at Paxata. “Meaning if I tried something and it doesn’t give me good [results]…I want to be able to go back and not throw away all of the things that I’ve done.” In this respect, provenance is more than a history of what’s happened to data, but an “ability to move up and down, back and forth on the lineage of that data and re-look at it two weeks, or two months, or six months down the line, [which] is absolutely pivotal because this is where your insight creations happen,” Loubser said.
Data quality implications
Increased data quality is one of the most desirable outputs for data governance. It can help decrease enterprise risk, perhaps the penultimate objective of effective data governance. In some ways, most of the automation measures discussed herein produce some effect on data quality, whether positive or negative. Automated agents may accelerate data processing, but the timely application of human-in-the-loop (via gamification technologies) to oversee their efforts is crucial to ensuring quality results.
Impact analysis approaches to regulatory compliance (collectively and for specific datasets) require the same oversight. Human validation of the automated classification techniques detailed is also necessary, while the active data provenance measures referenced offer a means of revising automation efforts if needed—as well as revisiting them and their downstream consequences. “It becomes very critical to have eyes on the data very, very quickly, because that’s when inside developments happen,” Loubser said.
Auditability
The nuanced relationship between data governance and automation is well worth examining. In many ways, it simply fortifies the need for human involvement (of data stewards, in particular) in a supervisory role based on validation, as opposed to rote processing. It’s not enough to simply govern individual models or technologies like machine learning, but to ensure their results are decreasing risk associated with data while increasing its enterprise value. Auditing will become an integral aspect of data governance as it marches into the New Year—and not just because regulators demand it.
“There’s all kinds of audits and various ways to do them in enterprises,” Coyne reflected. “There’s going to be audits of governance systems, audits of governance frameworks. Like okay, your governance framework seems to be working very well but how often do you check that everything really is? Especially these automated parts, do you do tests for that? Do you document what you find? It sounds very hairy, but that’s where things have to go.”
The sooner organizations realize these necessities and implement them, the more capable they’ll be when it comes to reaping the rewards of automation.
Jelani Harper is an editorial consultant servicing the information technology market, specializing in data-driven applications focused on semantic technologies, data governance and analytics.
About the Author(s)
You May Also Like