The Role of Quantum in the World of ChatGPT
Quantum computing-powered chatbots could learn from smaller datasets
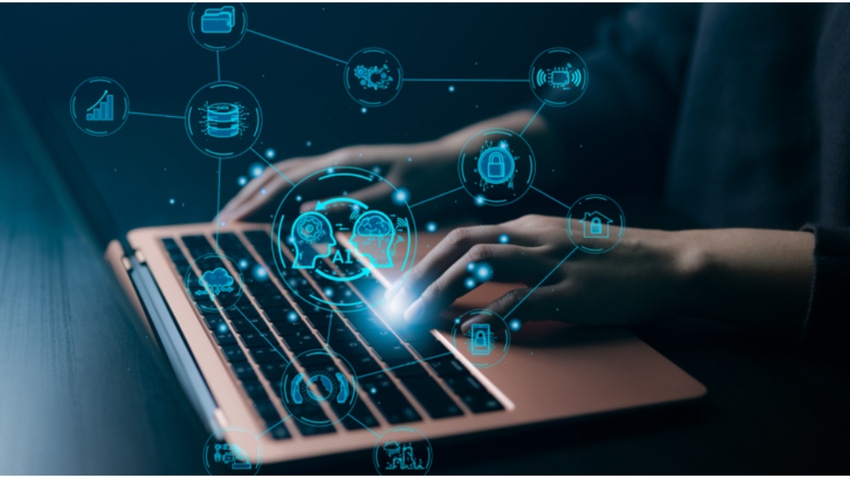
ChatGPT, the chatbot developed by OpenAI and launched late last year, is everywhere: writing poetry, telling jokes, giving relationship advice, explaining complex topics and cheating on school assignments.
It uses OpenAI’s large language models (LLMs), language processing techniques that enable computers to understand and generate text. They are trained by going through billions of pages of material to pick up context and meaning.
ChatGPT is an example of generative artificial intelligence (AI), which describes algorithms that can be used to create new content, including audio, code, images, text, simulations and videos.
Part of the process is natural language processing (NLP), which combines linguistics, computer science and artificial intelligence to understand and mimic how humans use language.
Quantum computing is already proving its worth in improving AI by discovering patterns in large, complex datasets.
Sam Lucero, chief quantum computing analyst at tech analyst and consultancy firm Omdia, sees a role for quantum computing in NLP and, ultimately in ChatGPT and generative AI in general. There is already a branch of study called quantum NLP, or QNLP.
Lucero cites two potential benefits.
“The first is being able to utilize a much larger ‘search space’ to find a solution,” he says.
“Practically speaking, this means QNLP could be much better at working with idiomatic language, for example, or better able to translate in cases where parts of speech in one language are structured very differently from the second language.”
The second potential benefit is it could be dramatically more efficient in training, needing much less training data to achieve the same level of ability.
“This could be key because large foundational models are apparently growing faster in size than Moore’s Law – so issues of cost, energy consumption, data availability and environmental impact become a concern,” Lucero says.
“There could also be an interesting enterprise angle from the standpoint of being able to train on the enterprise’s relatively smaller base of data – compared to, say, the internet – while achieving similar inferencing capabilities on the other end.”
However, like many potential applications for quantum computing, a practical solution could be years away.
“These benefits are theoretical at the moment, but not achievable in a way that offers an absolute advantage over classical NLP yet,” Lucero says.
“The closest to an ‘advantage’ announcement in generative AI comes from (enterprise quantum software company) Zapata, but they’re careful to state only an advantage against the classical algorithms that would typically be used, not against any possible classical algorithm. In their specific case, the generative AI approach was to develop a stock portfolio recommendation that delivered better returns relative to a fixed risk profile, not QNLP.”
This article is from sister publication Enter Quantum. To get the latest Quantum news, research, videos and other content, sign up for EQ's newsletter.
Read more about:
ChatGPT / Generative AIAbout the Author(s)
You May Also Like