To err is human, to never err is responsible AI
Human biases are one of the most common causes of ethical issues that plague AI systems
November 30, 2020
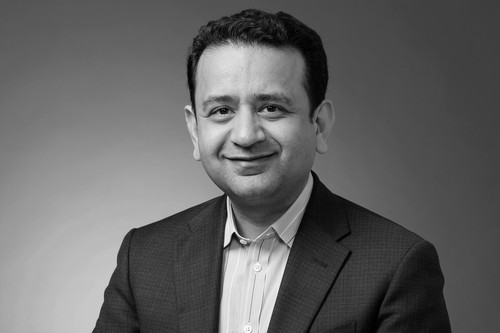
Sponsored Content
If to err is human, can we say never to err is AI? The blunder by an AI camera deployed at the club of Scottish soccer team Inverness Caledonian Thistle FC, of mistaking the lineman’s bald head for the soccer ball was, hilarious, but it certainly wasn’t funny for AI enthusiasts.
Especially when AI fuzziness leads to serious accidents or discrimination, technologists need to worry about it.
Hollywood buffs will surely not forget the 2004 Will Smith starrer ‘I, Robot’ movie that picturized a robot saving a police officer and allowing a child to drown based on ‘AI’ logic.
The great benefits that AI promises have propelled large-scale adoption of the technology. The global business spending on AI is expected to reach $50 billion in 2020 and $110 billion annually by 2024, despite pandemic-induced global economic slump as per an August 2020 IDC analyst report. AI will soon become part of every human life. Are we paying adequate attention to the hazards of allowing ‘artificial’ intelligence to run our lives? Are we building responsible AI?
World over, government bodies and international organizations are creating frameworks that define and dictate the creation and use of AI. Amongst these, Europe has demonstrated a strong play in building a ‘human-centric’ approach to AI by focusing on building a formal regulatory framework for the ethical use of AI. What do these policies mean at the ground level, particularly to companies providing AI services or building AI-based platforms and applications?
Human biases are one of the most common causes of ethical issues that plague AI systems. AI stupidity, misuse of AI, orchestration of human behavior via AI are other concerns. Let us examine some of the elements that must be considered when building AI-based solutions.
Creating a balance between augmenting human capabilities and ethical dangers of privacy and data misuse
AI is typically used to augment human abilities. From helping make optimal decisions to enhancing skills. This implies that AI-based systems need to access information about individuals leading to questions about data privacy. Another challenge is the lack of view into the biases that may exist within the vast amount of data that algorithm designers use to build AI systems. The data is often generalized and can result in skewed outcomes. There is also the possibility of fabrication of information with an intent to manipulate. It could lead to misuse of data to target specific user groups for unfair social practices such as racial discrimination.
Such ethical dangers can be addressed by creating international standards and guidelines such as the GDPR, building solutions around fraud detection and scrutiny, and bringing human intervention where required. Building trust also becomes important to ensure users are comfortable sharing their data and are aware of the processes or the uses to which it will be used.
Removing bias through data evaluation, algorithm audits, and re-training
Bias in AI comes from humans who pass along their prejudices in the training data they provide or in the models they build. Amazon had to do away with its recruiting tool that showed bias against women. A Twitter account run by Microsoft chatbots had to be shut when its algorithm ‘taught’ itself to post tweets that smacked racism. A pragmatic evaluation of bias is essential to ensure the ‘right set of data’ is made available for AI systems to ‘learn’ from. Differential privacy is a new approach that includes adding random noise into the data mix so that the resulting algorithm can be difficult to crack even for hackers who have access to auxiliary information. While bias detection and mitigation algorithms are available to balance the data, the best way to detect any anomaly is still through human intervention, which unfortunately cannot be scaled.
The most effective ML algorithms are those that can self-learn and improvise based on fresh input from real-data. Once the algorithm is developed, it should be tested and re-trained based on the results to ensure bias is avoided without impacting the predictive capability of the algorithm. Lastly, it is most important to have a multi-disciplinary, research-based approach to building algorithms.
Building explainability through a multi-disciplinary approach
Explainability is about understanding how an AI model came to its conclusions about a particular input scenario. How intelligent, transparent, and explainable is the AI system? How much confidence can we place on its outcome? For example, how did an autopilot sensor in a Tesla car fail to detect a white colored trailer across a bright sky?
Explainability needs to be defined for three stakeholders – system designers to understand the scope of operation of the models, decision-makers to understand factors that might have contributed to the decision and ensure that such factors are relevant, and the end-user in terms of trustworthiness, fairness and impartiality.
An AI-based model should provide decision support to a human by providing recommendation based on a problem analysis along with a certainty measure that reflects the confidence the system has in the particular analysis. It should be able to point out to the input data used as evidence to reach the said conclusion, while revealing the reason behind the exclusion of various alternative possibilities.
Spreading data-risk awareness and guarding against security risks
As with most digital technologies, a single breach or hacking incident can jeopardize data and thereby the outcomes of an AI implementation. Also, by sending a pre-meditated series of queries to an AI model it is possible for a hacker to reverse engineer sensitive information in the training dataset. To summarize, AI models created from a sample set of data makes certain general assumptions about the data leaving potential blind spots in the algorithm, which adversaries could notice and take advantage of. In such cases, identifying these weak spots and implementing security and privacy best practices becomes a mandate.
The above best practices will help create the framework required to deliver AI capabilities within the ethical frameworks and policies as defined by governments and corporates and thereby ensure organizations use AI for the greater benefit of human society with minimum risks to human values.
The above best practices will help create the framework required to deliver AI capabilities that are within the ethical frameworks and policies as being defined by governments and corporates and thereby ensure organizations use AI for the greater benefit of the human society with minimum risks to human values. I am personally convinced that AI can significantly augment the quality of our lives, it just requires a strong focus by leaders across spectrums to manage such nuances to ensure it works to our collective advantage.
Mohit Joshi is president and head of Banking, Financial Services & Insurance, Healthcare and Life Sciences at Infosys.
You May Also Like