Machine vision could help develop components for nuclear reactors, suggests research from UMich
Startup Theia Scientific wants to launch pre-production of its defect detection unit by 2022
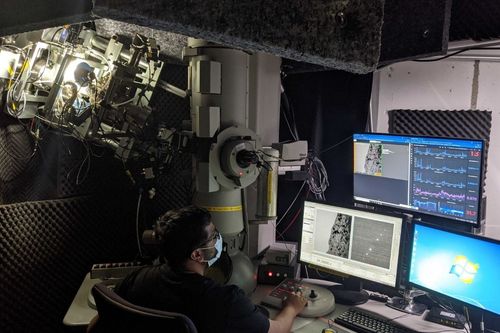
Startup Theia Scientific wants to launch pre-production of its defect detection unit by 2022
Machine vision has been used to detect tiny defects caused by prolonged exposure to radiation, in an experiment at the University of Michigan.
The system “could speed up the development of components for advanced nuclear reactors, which may play a critical role in reducing greenhouse gas emissions to fight climate change,” the announcement reads.
"We believe we are the first research team to ever demonstrate real-time image-based detection and quantification of radiation damage on the nanometer length scale in the world," said Kevin Field, an associate professor of nuclear engineering and radiological sciences and vice president of the machine vision startup Theia Scientific.
"We see clear pathways to accelerate discoveries in the energy, transportation, and biomedical sectors.”
Gone fission
The system works by sending beams of charged atoms—known as ions—at material samples. In the experiment at the Michigan Ion Beam Laboratory, the lab emulated the damage sustained after years or decades of use in a nuclear reactor.
An ion beam of the noble gas krypton was used to test samples of iron, chromium, and aluminum—a radiation-tolerant material of interest for both fission and fusion reactors.
The krypton ions created radiation defects – which appeared as planes of missing or extra atoms sandwiched between two ordinary crystal lattice planes.
The lab was then able to observe the development of these defects, appearing as black dots in the electron microscope images. The machine vision-powered system was used to detect and quantify radiation-induced defects instantaneously during the experiment.
The results were then displayed as graphics on the electron microscope imagery – with defects labeled, providing information on their size, number, location, and density.
"The real-time assessment of structural integrity allows us to stop early if a material is performing badly and cuts out any extensive human-based quantification," Field said. "We believe that our process reduces the time from idea to conclusion by nearly 80 times."
"Previously, we would record the whole video for the irradiation experiments and then characterize just a few frames," said Priyam Patki, a postdoctoral researcher in nuclear engineering and radiological sciences who ran the experiment with Field.
"But now, with the help of this technique, we are able to do it for each and every frame, giving us an insight into the dynamic behavior of the defects—in real time."
Pre-production units in 2022
The project was funded by the US Department of Energy’s Small Business Innovation Research Program.
Theia Scientific, the startup behind it, is working on a proposal to continue its research efforts, with pre-production detector units expected to be available in 2022.
Field co-founded Theia in 2020, utilizing datasets developed at the University of Michigan. Other collaborators on the project include Kai Sun, an associate research scientist in materials science and engineering; Fabian Naab, a research lab specialist in nuclear engineering and radiological sciences; and Dane Morgan, a Professor of Engineering at the University of Wisconsin.
About the Author(s)
You May Also Like