McKinsey: AI Adoption Plateaus But Usage of Capabilities Doubles
A quarter say at least 5% of earnings is attributable to AI use
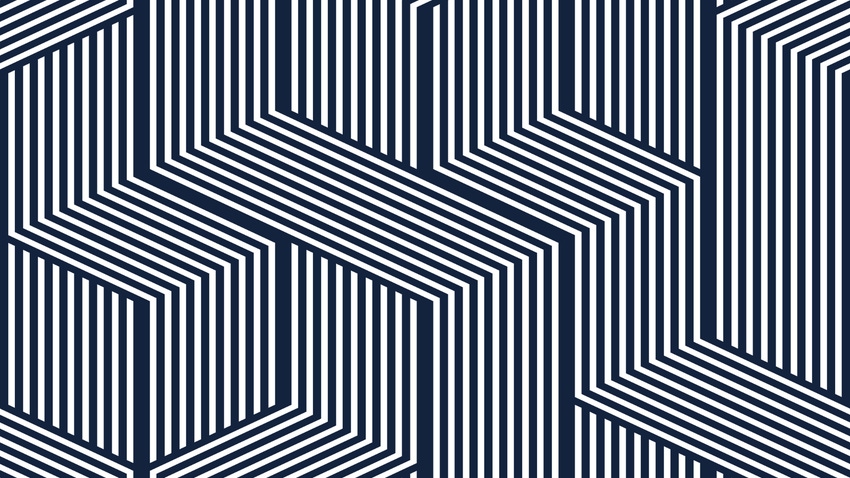
The percentage of companies adopting AI has stayed steady over the last five years, but their use of AI capabilities has doubled, according to the latest McKinsey report.
In 2017, 20% of companies surveyed adopted AI in at least one business area. This year, 50% of firms are deploying AI and the level has stayed in the range of 50% to 58% since 2019, according to the McKinsey Global Survey on AI.
However, companies’ use of AI capabilities has taken off. The average number of capabilities that have been embedded within at least one function or business unit rose from 1.9 in 2018 to 3.8 this year. Top capabilities are robotic process automation and computer vision while NLP came in third, advancing from the middle of the pack.
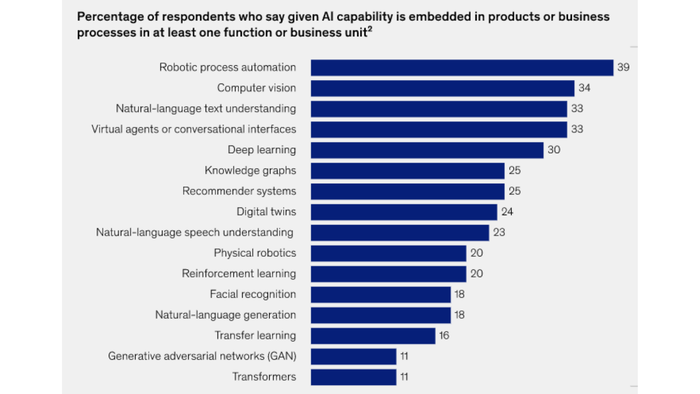
The report noted that the top use cases have stayed fairly constant in the past four years: Optimization of service operations continues to lead the chart. Second is creation of new AI-based products, then customer service analytics, customer segmentation and new AI-based enhancements of products.
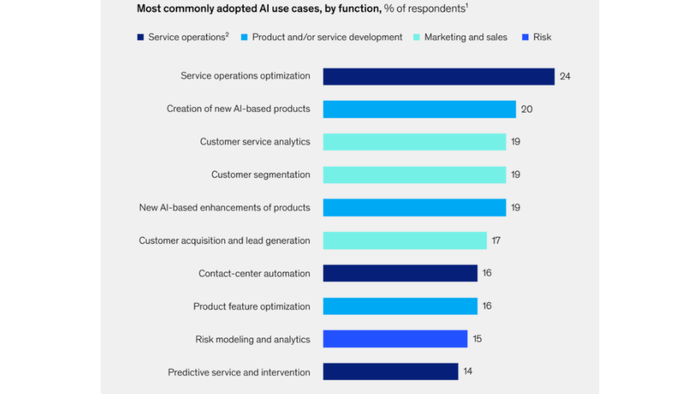
Furthermore, AI investment has skyrocketed. In 2017, 40% of the respondents said that over 5% of their digital budgets were earmarked for AI. That’s now up to 52% of respondents saying the same thing in 2022. Looking ahead to the next three years, 63% believe their companies will boost AI investment even more.
Where companies see value from AI also has changed. In 2018, risk and manufacturing were seen as the two areas where companies saw value. In 2022, the biggest revenue gains are in marketing and sales, product and service development, and strategy and corporate finance. As for the area with the highest cost benefit from AI? It is supply chain management.
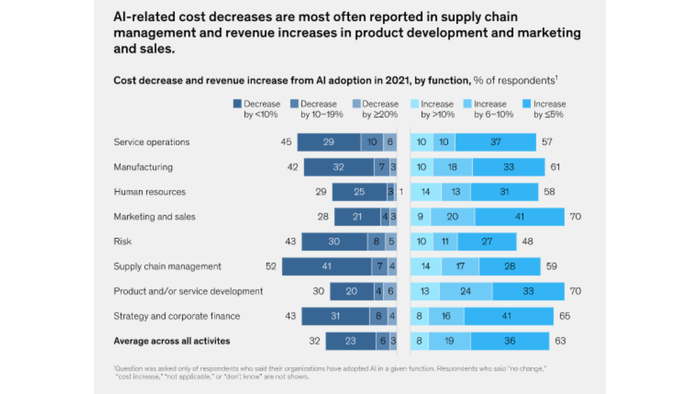
In the past half decade, “we have seen the ‘AI winter’ turn into an ‘AI spring’,” wrote Michael Chui, partner at McKinsey Global Institute, in the report. But after a period of “initial exuberance, we appear to have reached a plateau. … We might be seeing reality sinking in at some organizations of the level of organizational change it takes to successfully embed this technology.”
Chui said some companies have gotten discouraged “because they went into AI thinking it would be a quick exercise.” Others have made steady progress because they took the long view and transformed into learning organizations to build their “AI muscles” first.
He added that there is an “emerging playbook for getting maximum value from AI.”
Playbook for success
For about a quarter of companies, at least 5% of their earnings before interest and taxes (EBIT) can be attributed to AI. “The bottom-line value realized from AI remains strong and largely consistent,” the report said.
Among AI leaders, the profit lift came more from revenue gains than cost reductions.
What are they doing right? Their best practices include linking AI strategy to business outcomes and engaging in ‘frontier’ practices to shift from experimenting with AI to industrializing it, according to Bryce Hall, associate partner at McKinsey.
Examples are machine learning operations practices such as assetization, or turning things like code into reusable assets that can be reapplied to different business applications. Also, leaders often develop a data architecture that is modular enough to accommodate new AI applications quickly.
Moreover, they automate most data-related processes to improve “efficiency in AI development and expand the number of AI applications they can develop by providing more high quality data to feed into AI algorithms.”
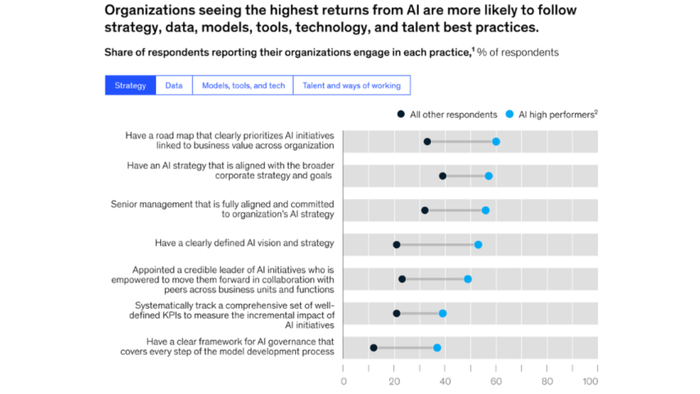
High performers were 1.6 times more likely to use low-code, no-code programs to engage their non-technical employees in creating AI applications. This speeds up development of these applications.
High performers also were more likely to follow specific scaling practices, such as “using standardized tool sets to create production-ready pipelines and using an end-to-end platform for AI-related data science, data engineering, and application development” they created in-house.
Leaders were more likely to mitigate risk by ensuring AI and data governance, standardizing processes and protocols, automating processes such as data quality control to remove errors introduced manually, and testing validity of models and monitoring them over time.
Risks and hiring woes
However, one concern is that as the use of AI capabilities has soared, actions taken to mitigate risk have not kept pace since 2019. These AI-related risks encompass regulatory compliance, cybersecurity, privacy, explainability, organizational reputation, equity and fairness, workforce displacement, physical safety, national security and political stability.
Hiring remains a continuing challenge as well in an expanding AI market. While software engineers and data engineers have topped the hiring charts, AI data scientists are the hardest to find. Organizations have turned to upskilling and reskilling as an alternative to fill in the gaps.
The McKinsey report garnered responses from nearly 1,500 participants of which half had adopted AI. These AI practitioners were asked about their companies' use of AI.
About the Author(s)
You May Also Like